Table of Contents
Understanding Federated Learning in Ad Tech
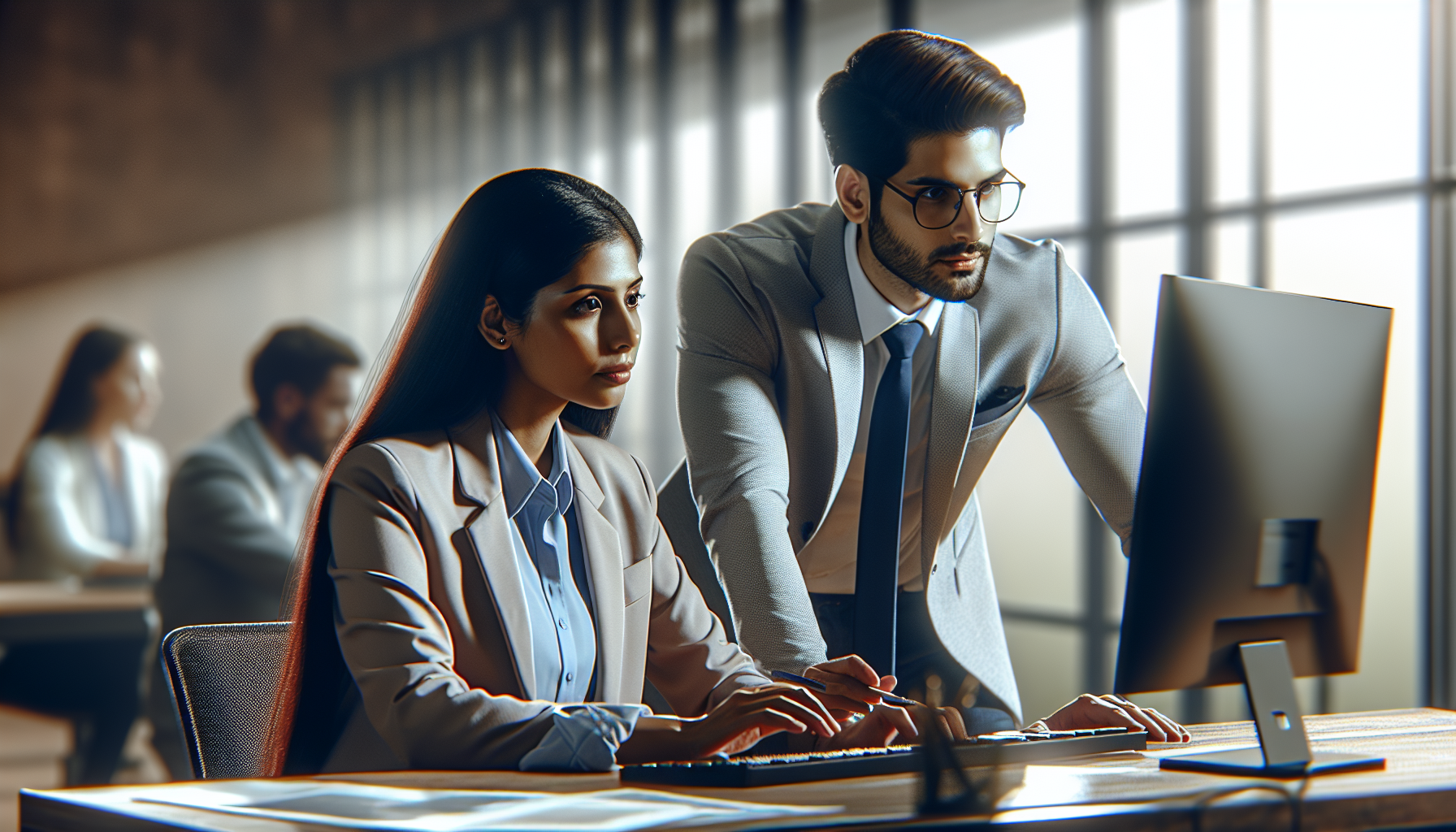
Key Points
- Federated learning allows multiple devices to contribute to machine learning models without sharing the data itself, enhancing privacy.
- This method is particularly relevant as privacy regulations tighten and traditional data aggregation becomes more challenging.
- It involves a decentralized approach where updates to the model are aggregated without transferring raw data from the devices.
- Federated learning can be implemented in both centralized and decentralized architectures, depending on the specific requirements and constraints of the application.
- While it offers enhanced privacy, federated learning does not completely eliminate the risk of data exposure but significantly reduces it.
Technical Overview
Federated learning involves training algorithms across multiple decentralized devices or servers holding local data samples without exchanging them. This process protects users’ privacy by design, as sensitive data does not leave their devices. Instead, only model updates are shared with a central server, where they are averaged and redistributed to improve the model iteratively. This technique is particularly useful in environments where data privacy is paramount, such as in personalized advertising and healthcare. By leveraging local computations, federated learning enables a collaborative model that benefits from the collective insights of all participating devices while keeping each dataset secure and private. The central server plays a crucial role in this setup. It aggregates the updates received from all participants and applies them to the global model. The improved model is then sent back to the participants for further training. This cycle continues until the model reaches an optimal level of accuracy. Importantly, federated learning requires robust network connections and sophisticated algorithms to manage the distribution and aggregation of model updates. This can introduce challenges in synchronization and scaling, particularly with a large number of participants.Applications in Digital Advertising
In the context of digital advertising, federated learning enables advertisers to create personalized ad experiences without compromising user privacy. By processing data locally on users’ devices, advertisers can target ads based on user behavior without needing to know the specifics of that behavior. This method is a response to increasing user concerns and regulatory demands regarding privacy. It allows for the continuation of personalized advertising practices while adhering to privacy laws and norms. Federated learning thus represents a shift towards more sustainable and privacy-conscious advertising strategies. Moreover, federated learning can improve the efficiency of ad targeting algorithms by enabling them to learn from a broader range of data inputs without compromising on privacy. This can lead to more effective advertising campaigns that are better tailored to the preferences of diverse user bases. Overall, the integration of federated learning into digital advertising frameworks offers a promising path forward in the balance between personalized marketing and privacy preservation.The Challenge of Data Privacy in Ad Targeting
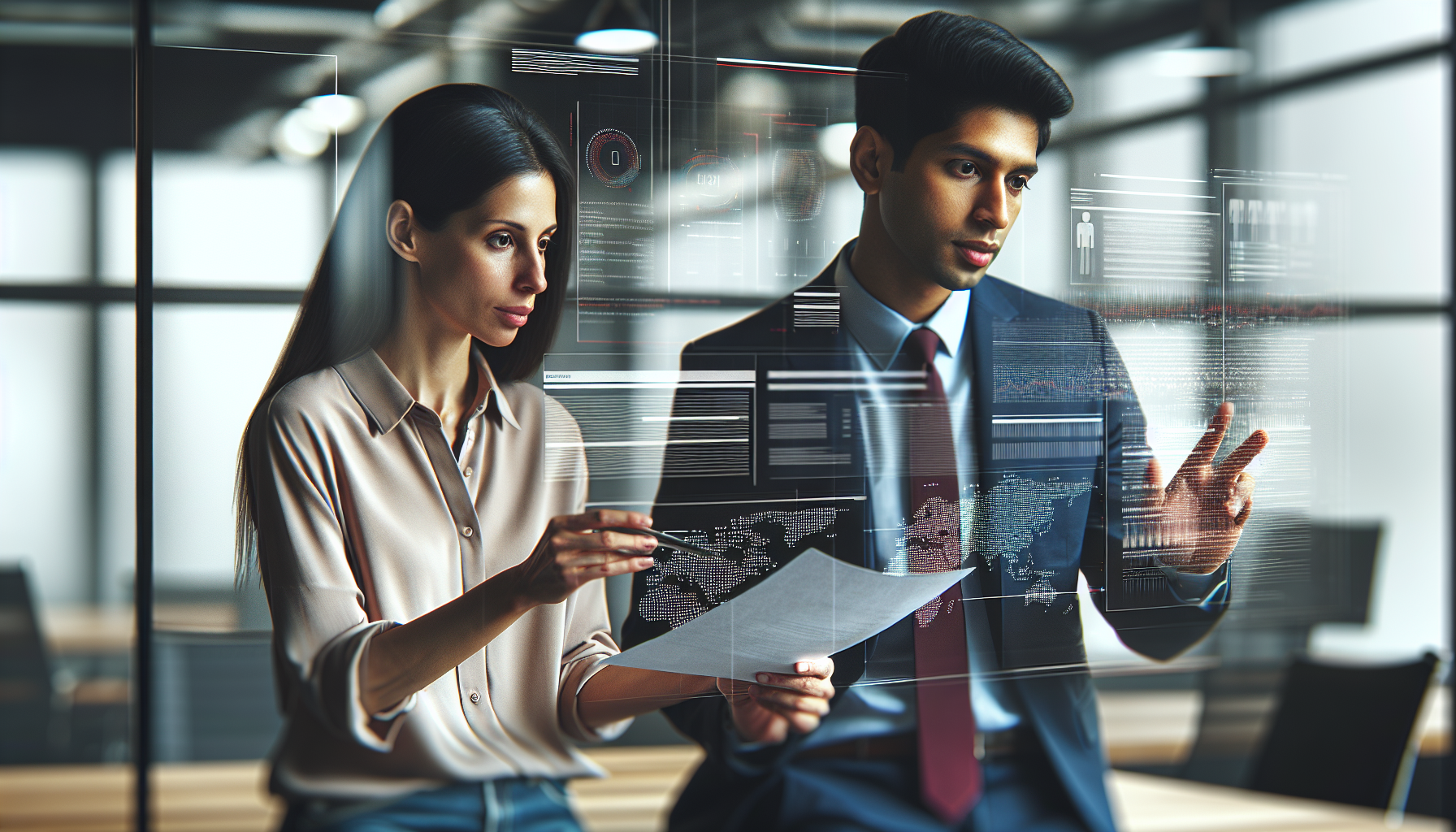
Real-World Implications
One of the most pressing challenges in today’s advertising technology landscape is maintaining user privacy while delivering effective ad targeting. Traditional methods often involve collecting vast amounts of personal data, leading to privacy concerns and regulatory scrutiny. As regulations like the General Data Protection Regulation (GDPR) and the California Consumer Privacy Act (CCPA) impose stricter data handling requirements, ad tech companies must innovate to stay compliant while still achieving their marketing goals. This situation creates a complex environment where the need for advanced solutions like federated learning becomes apparent. The challenge is not just technical but also ethical, as companies must navigate the fine line between effective marketing and respect for user privacy. The integration of privacy-preserving technologies such as federated learning is, therefore, not only a technical requirement but also an ethical imperative in the modern digital advertising ecosystem. Addressing this challenge requires a deep understanding of both the technological aspects of federated learning and the regulatory landscape governing data privacy. This dual focus ensures that solutions are not only effective but also compliant with global privacy standards.Implementing Federated Learning for Enhanced Privacy
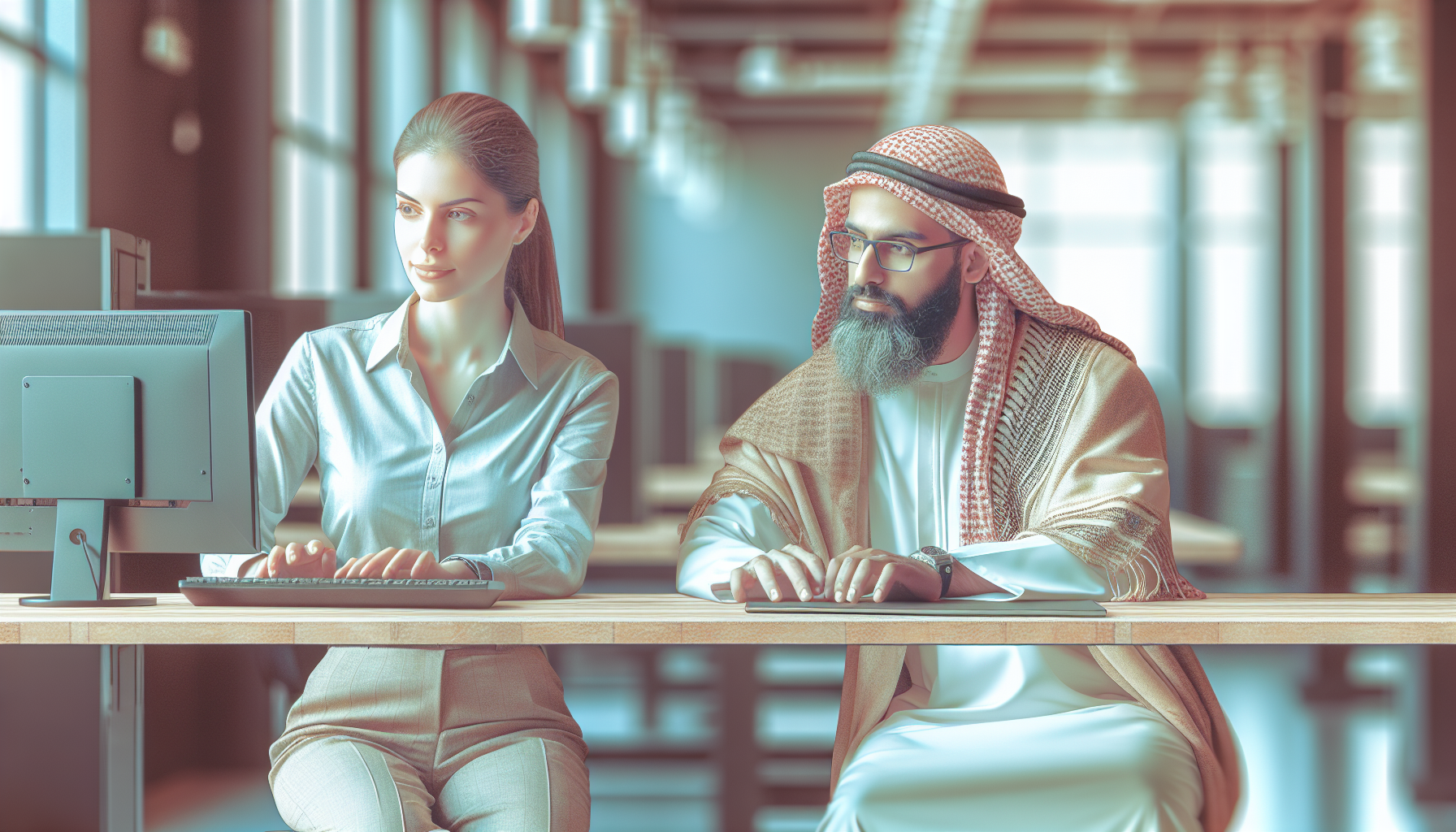
Step-by-Step Approach
- To integrate federated learning into an ad targeting system, start by identifying the specific needs and constraints of your advertising operations. This includes understanding the data types involved, the privacy requirements, and the desired outcomes of your ad campaigns.
- Next, design a federated learning system tailored to these needs. This involves setting up a central server to coordinate the model training and ensuring that all participating devices are capable of performing the necessary computations.
- Develop the algorithms that will run on users’ devices. These algorithms should be designed to learn from user data locally and generate updates to the model without transmitting sensitive information.
- Finally, a robust communication protocol between the central server and the participating devices must be implemented. This protocol must ensure secure and efficient transmission of model updates while minimizing the risk of data leakage.
Frequently Asked Questions
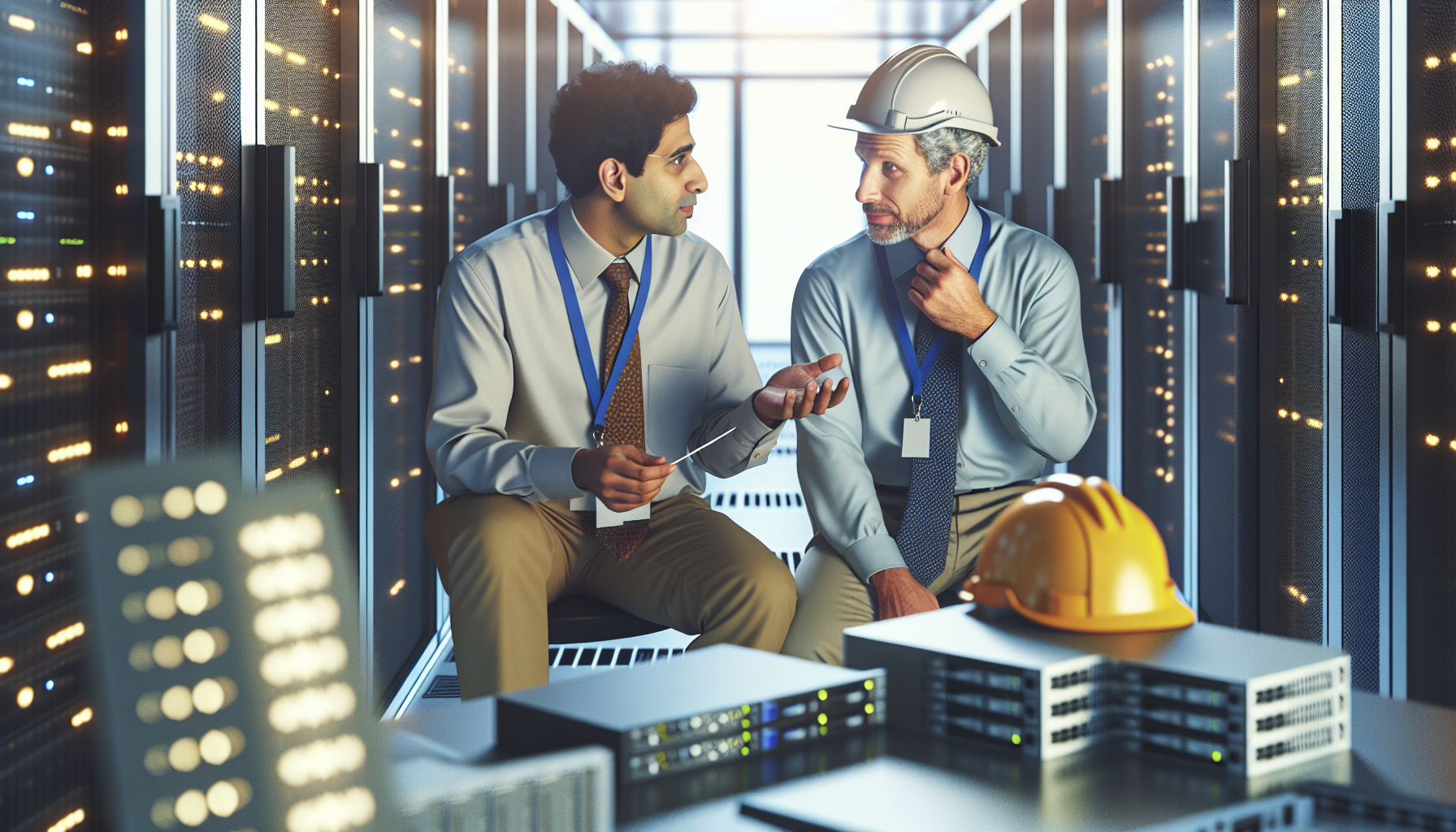
What is federated learning?
Federated learning is a machine learning technique that trains an algorithm across multiple decentralized devices or servers without exchanging the data samples, thus maintaining data privacy.How does federated learning enhance privacy?
By processing data locally on each device and only sharing model updates, federated learning minimizes the risk of exposing sensitive user data.Can federated learning be used in industries other than advertising?
Yes, federated learning is applicable in various sectors, including healthcare, finance, and smart manufacturing, where data privacy is crucial.What are the challenges of implementing federated learning?
Challenges include managing communication and data synchronization across numerous devices, ensuring robust security measures, and handling computational disparities among devices.Is federated learning the ultimate solution for privacy in ad tech?
While federated learning significantly enhances privacy, it is part of a broader set of solutions needed to address all aspects of data security and regulatory compliance in advertising.Looking Ahead: The Future of Federated Learning in Ad Tech
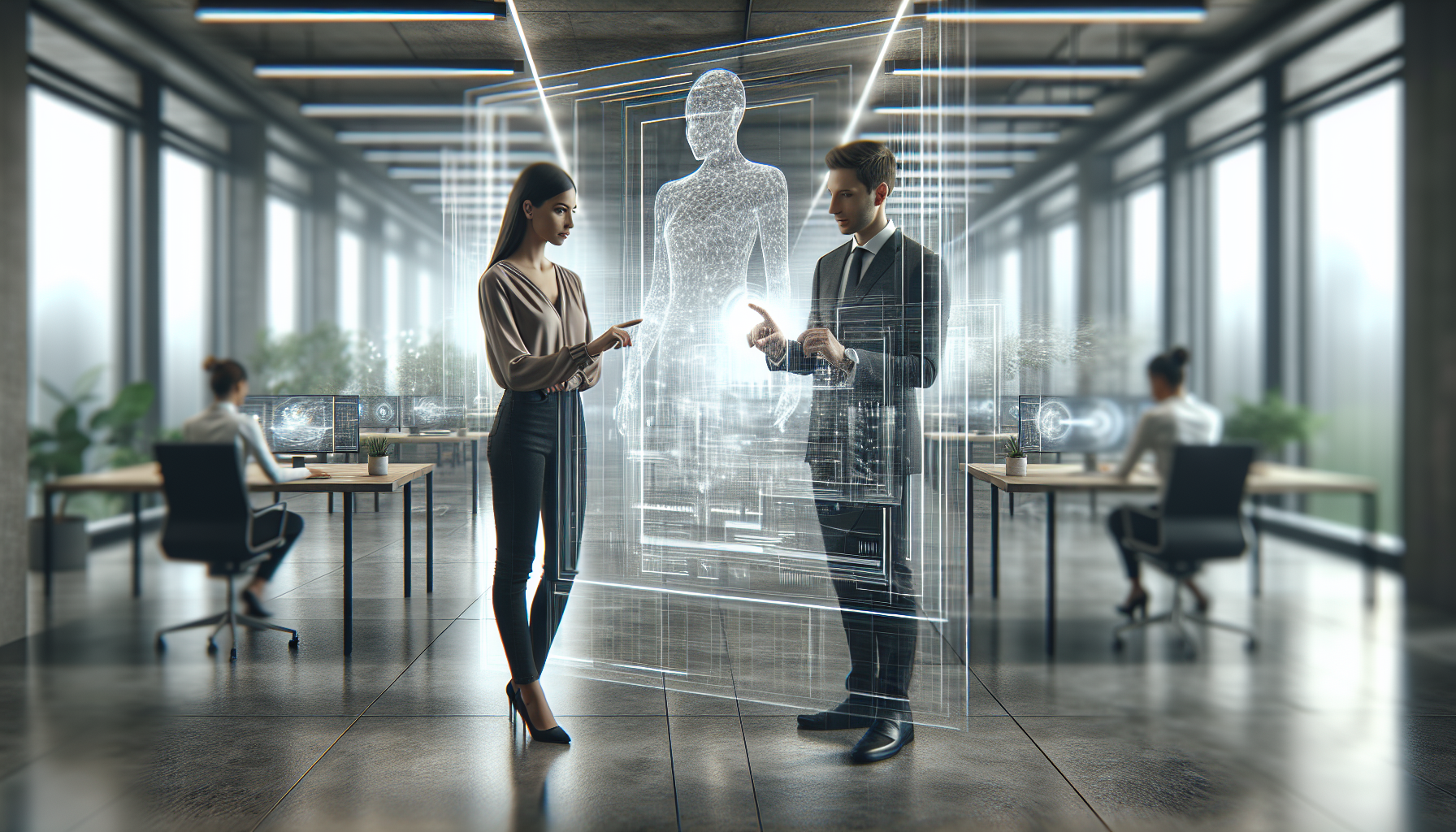
- Increased Adoption: As privacy concerns grow, more companies will turn to federated learning as a viable solution for maintaining user trust while delivering personalized experiences.
- Regulatory Influence: Global data protection regulations will drive the adoption of privacy-preserving technologies like federated learning in digital advertising.
- Technological Advancements: Continued advancements in machine learning and data processing will enhance the efficiency and effectiveness of federated learning models.
- Expansion into New Markets: Federated learning will find new applications in emerging markets and industries, broadening its impact beyond traditional sectors.
- Integration with Other Technologies: Federated learning will increasingly be integrated with other privacy-enhancing technologies to create comprehensive solutions for data privacy.
References
- Federated Learning of Cohorts on Wikipedia: A comprehensive overview of the Federated Learning of Cohorts, detailing its function and implications in privacy-preserving technologies.
- What is Federated Learning in digital advertising? | Mobile Dev Memo: An article that discusses the application of federated learning in the digital advertising space.
- Building a privacy-first future for web advertising | Google Blog: Insights into Google’s initiatives for enhancing privacy in web advertising, including the use of federated learning.
- Federated Learning and Consumer Data | Apheris AI: An exploration of the opportunities federated learning presents in utilizing consumer data while preserving privacy.
- What is Google FloC? Federated Learning of Cohorts Explained | AdTech Explained: This is a detailed explanation of Google’s Federated Learning of Cohorts and its impact on advertising technology.
Disclaimer
This article is AI-generated for educational purposes and does not intend to provide advice or recommend its implementation. The goal is to inspire readers to research and delve deeper into the topics covered.
Latest posts by Leo Celis (see all)
- From SaaS to AI Agents - 05/27/25
- The AI Automation Engineer - 05/13/25
- Hire One Developer to Press One Key - 05/06/25