Table of Contents
Overview of AI-Driven Threat Intelligence
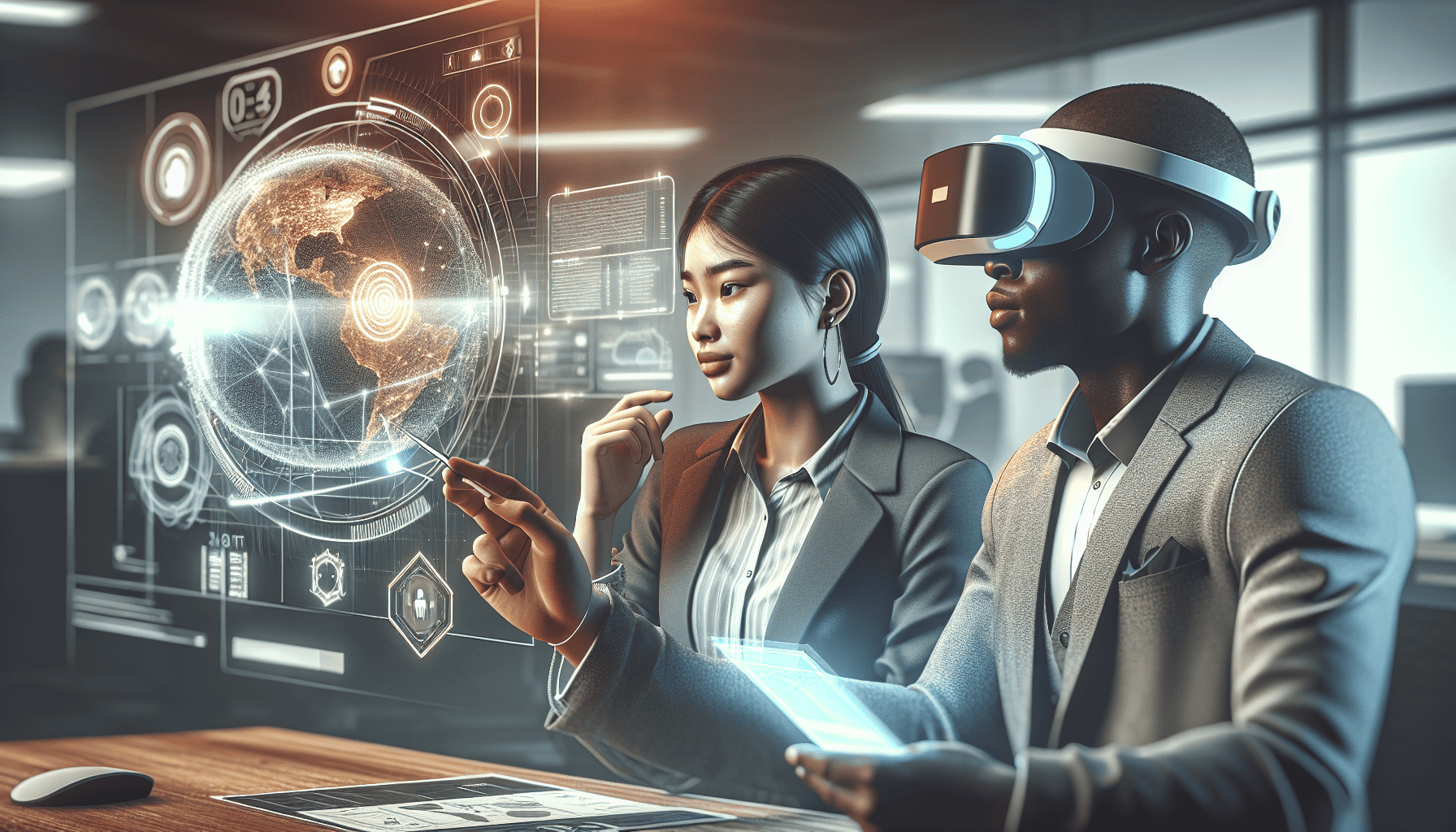
What is AI-Driven Threat Intelligence?
AI-driven threat intelligence refers to the use of artificial intelligence (AI) to analyze and predict security threats in real-time, particularly in complex networks like those found in Industrial IoT (IIoT). This technology leverages machine learning algorithms and big data analytics to detect anomalies and potential threats, enhancing the security posture of industrial systems. By integrating AI into cybersecurity frameworks, organizations can preemptively identify and mitigate risks before they escalate into serious breaches. This proactive approach is crucial in protecting critical infrastructure and sensitive data in industries such as manufacturing, energy, and transportation. Moreover, AI-driven systems continuously learn and adapt to new threats, ensuring that security measures evolve in tandem with emerging threats. This dynamic capability is essential for maintaining the integrity and resilience of industrial operations in the face of increasingly sophisticated cyber-attacks.Benefits of AI-Driven Threat Intelligence in Industrial IoT
The integration of AI-driven threat intelligence into Industrial IoT systems offers numerous benefits, including enhanced detection capabilities and improved operational efficiency. By automating the threat detection process, AI reduces the reliance on manual intervention, allowing security teams to focus on strategic response measures rather than routine detection tasks. Additionally, AI-driven solutions can analyze vast amounts of data much faster than human analysts, leading to quicker response times and minimizing the potential damage from attacks. This speed is particularly critical in industrial environments where even minor delays can lead to significant disruptions and financial losses. Furthermore, AI enhances the accuracy of threat detection by reducing false positives, which can drain resources and divert attention from genuine threats. Through continuous learning, AI models refine their understanding of what constitutes normal behavior within a specific industrial network, thereby improving their ability to flag actual anomalies.The Challenge of Securing Industrial IoT Networks
Complexity of Industrial IoT Systems
Industrial IoT networks are inherently complex, comprising various interconnected devices and systems that communicate over different protocols. This complexity not only makes the network difficult to manage but also increases its vulnerability to cyber-attacks. The diverse nature of devices, from sensors to high-end industrial controllers, each with its own firmware and software configurations, creates multiple points of entry for attackers. Securing each of these nodes individually and ensuring they comply with overall network security policies is a daunting task for IT managers.Increasing Sophistication of Cyber Threats
The sophistication of cyber threats targeting Industrial IoT systems is on the rise. Attackers are continually evolving their tactics, techniques, and procedures (TTPs), making traditional security measures insufficient. Advanced persistent threats (APTs), which are prolonged targeted attacks, can infiltrate networks undetected and cause significant damage over time. These threats often exploit zero-day vulnerabilities, for which patches or fixes are not yet available, complicating the defense strategies for industrial systems.Lack of Adequate Security Measures
Many Industrial IoT environments lack comprehensive security measures, partly due to the rapid deployment of IoT devices without proper consideration of security implications. This oversight leaves critical infrastructure exposed and vulnerable. Additionally, the integration of IT (Information Technology) and OT (Operational Technology) environments poses unique challenges. While IT security focuses on data confidentiality and integrity, OT security prioritizes system availability and safety. Balancing these priorities without compromising either side requires a nuanced approach that many organizations find difficult to implement effectively.Implementing AI-Driven Solutions
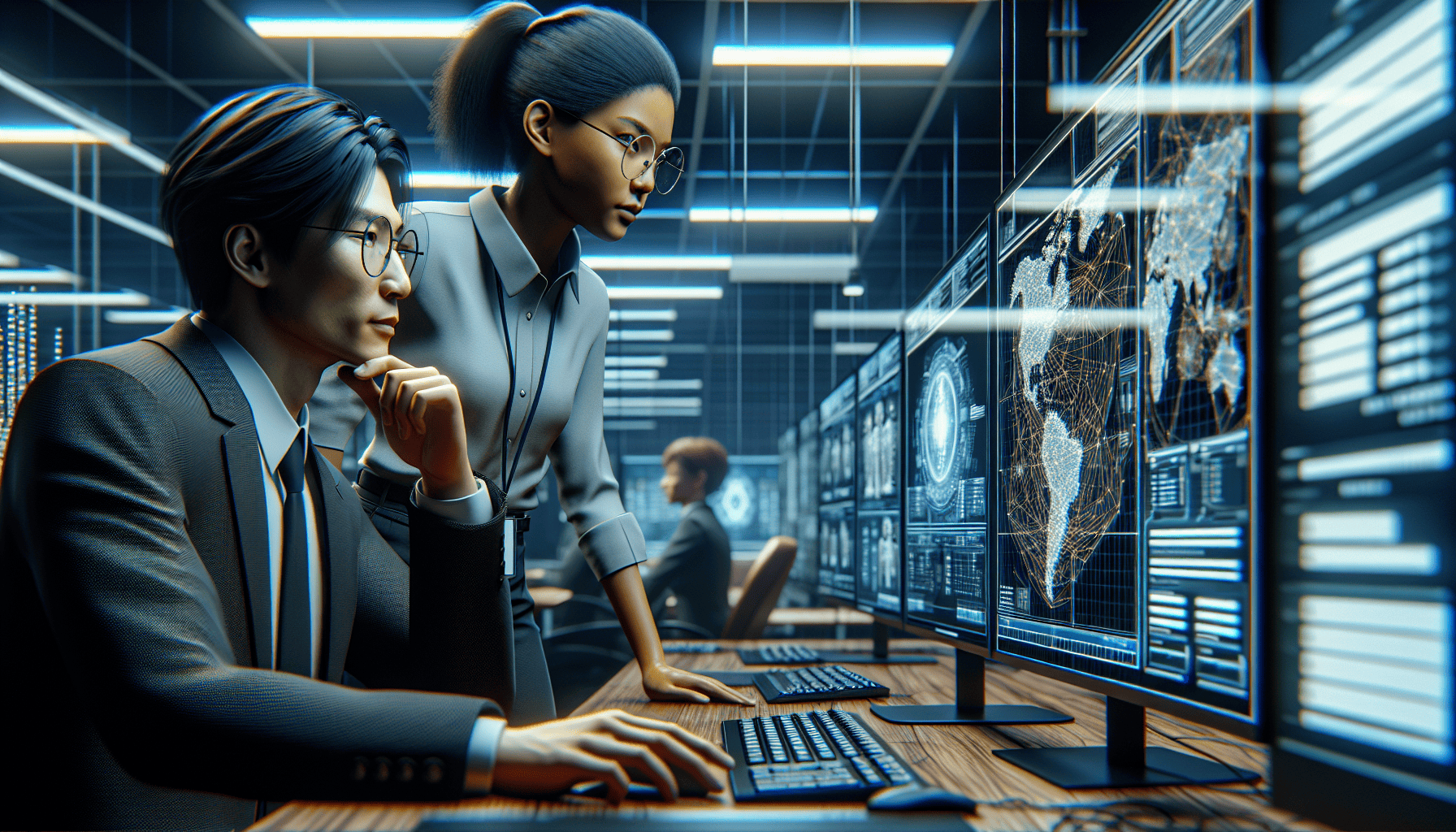
Step 1: Integration of AI Capabilities
Integrating AI capabilities into existing security infrastructures is crucial to addressing the cybersecurity challenges in industrial IoT. This involves deploying AI-powered tools that can analyze network traffic and detect anomalies indicative of a cyber threat. These tools should be capable of real-time analysis to provide immediate alerts and enable swift responses. The integration process also involves training the AI models on specific network behaviors to enhance their accuracy in detecting deviations from the norm.Step 2: Continuous Monitoring and Learning
Once AI tools are integrated, continuous monitoring of network activities is essential. AI-driven systems should be designed to learn from ongoing traffic and progressively improve their threat-detection capabilities. This learning process involves not just recognizing known threats but also adapting to new patterns that could indicate emerging risks. Continuous monitoring also helps in refining security policies and response strategies based on evolving threat landscapes.Step 3: Collaborative Threat Intelligence Sharing
The final step involves collaborative threat intelligence sharing among different stakeholders within the Industrial IoT ecosystem. By sharing insights about threats and vulnerabilities, organizations can enhance their collective defense mechanisms. This collaboration can be facilitated through platforms that allow secure sharing of threat data and response strategies. Such collective efforts are vital for staying ahead of cybercriminals and effectively protecting the interconnected digital and physical assets of Industrial IoT networks.Frequently Asked Questions
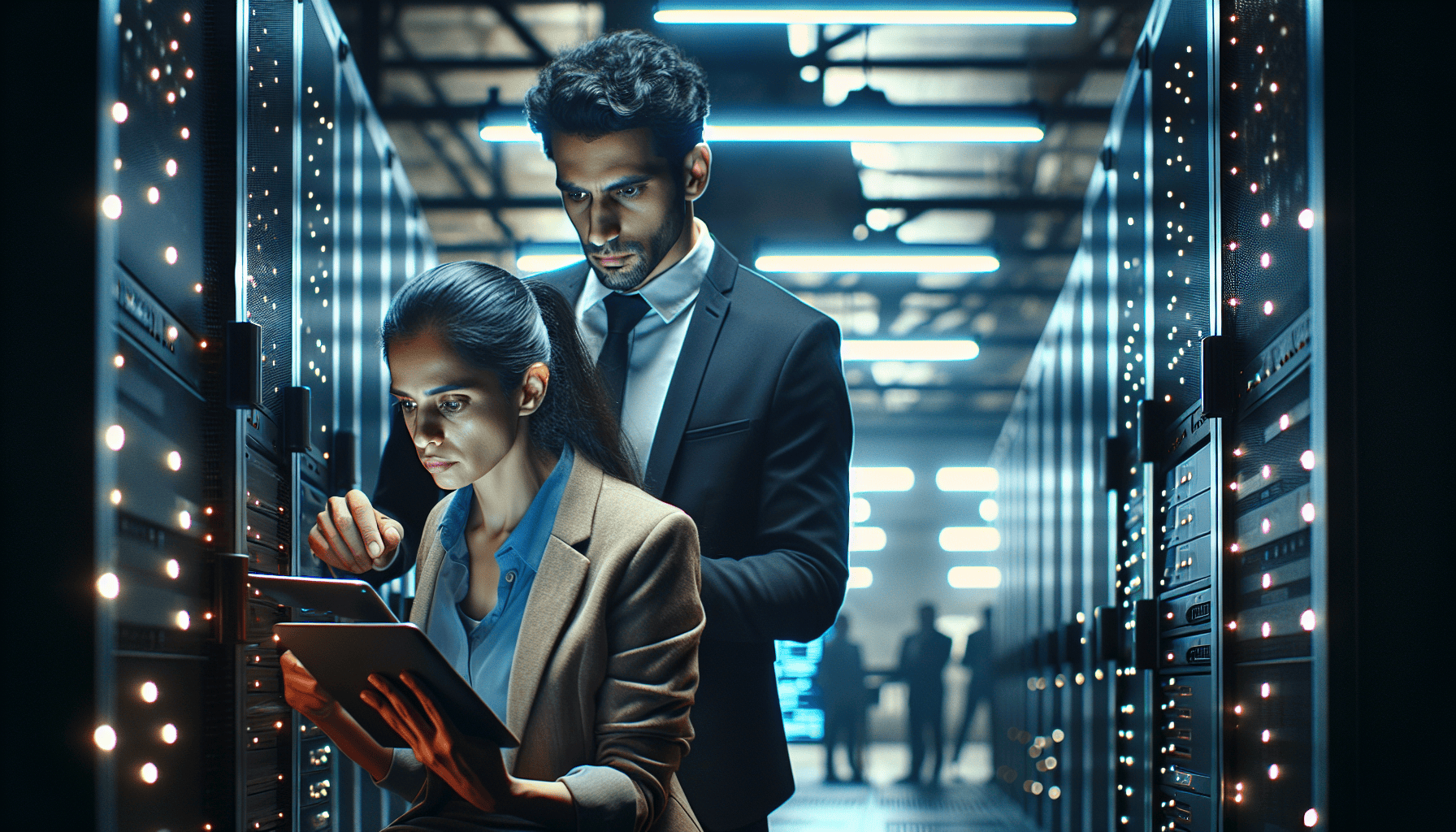
What is Industrial IoT?
Industrial IoT refers to the extension and use of the Internet of Things (IoT) in industrial sectors and applications. It involves the integration of digital and physical systems to enhance the automation and efficiency of industrial processes.How does AI enhance cybersecurity in Industrial IoT?
AI enhances cybersecurity by automating the detection of anomalies and potential threats, thereby enabling faster and more accurate responses. It helps manage the vast amount of data generated by IoT devices and identifies patterns that may indicate a security breach.What are the challenges of implementing AI in cybersecurity?
Challenges include the need for large datasets to train AI models, the integration of AI into existing security infrastructures, and the potential for AI-driven systems to generate false positives. Ensuring the AI system’s decisions are explainable and transparent is also crucial.Can AI-driven threat intelligence completely replace human analysts?
No, AI-driven threat intelligence is intended to augment human analysts, not replace them. AI can handle large volumes of data and perform routine tasks, but human oversight is essential for complex decision-making and response strategies.Looking Ahead: The Future of AI in Industrial IoT
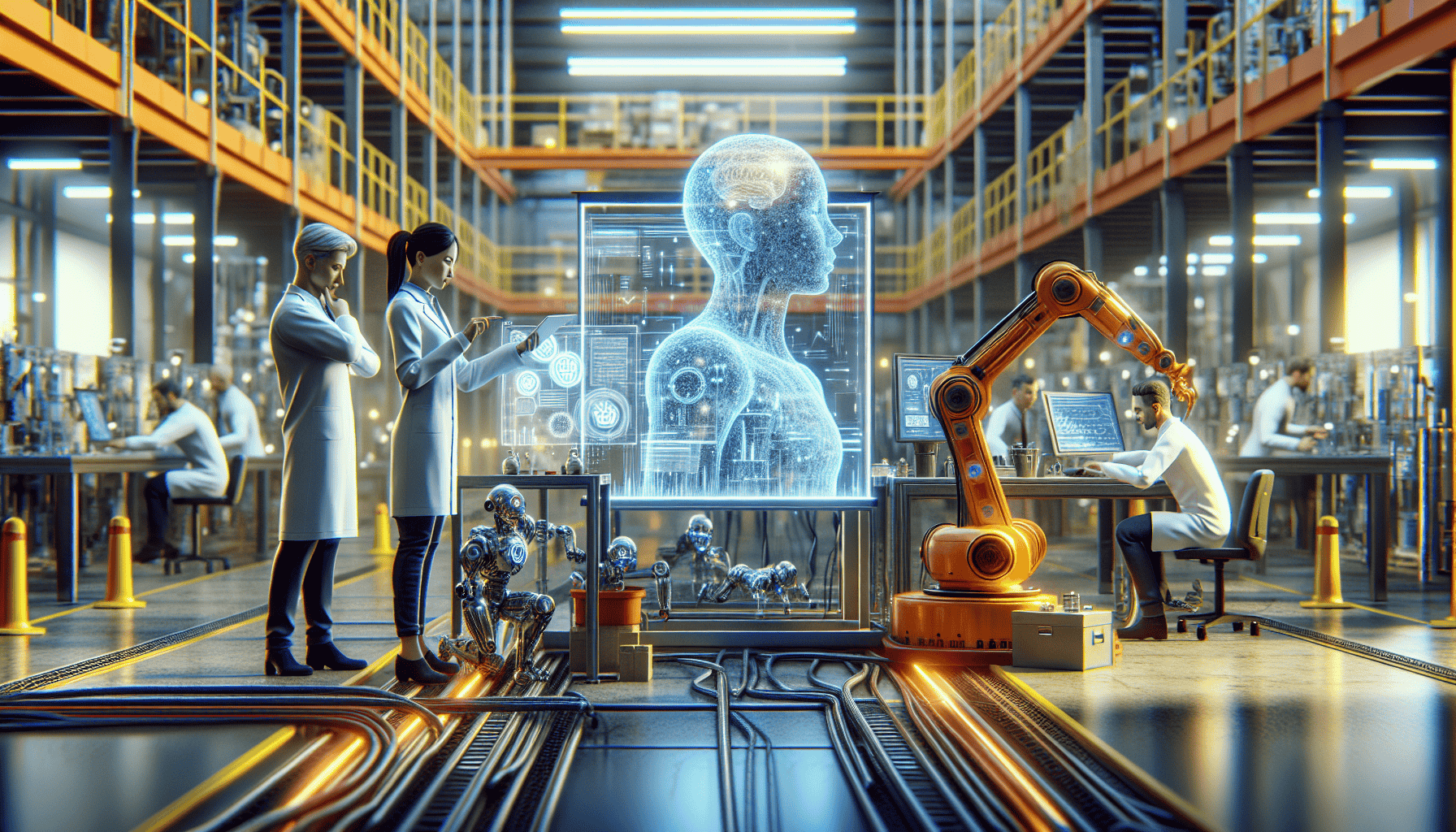
- Increased Adoption of AI As industries recognize the benefits, the adoption of AI in cybersecurity is expected to grow, leading to more sophisticated and resilient security solutions.
- Advancements in Machine Learning Improvements in machine learning algorithms will enhance the accuracy and efficiency of threat detection systems, reducing false positives and enabling real-time threat response.
- Greater Integration of IT and OT The convergence of IT and OT security practices will continue, driven by the need for comprehensive security frameworks that address the unique challenges of Industrial IoT.
- Expansion of Predictive Security AI will increasingly be used for predictive security, allowing companies to anticipate and mitigate potential threats before they manifest.
- Enhanced Collaboration Collaborative efforts in threat intelligence sharing will expand, leading to a more unified approach to cybersecurity across different sectors and regions.
More Information
- Industrial AI Framework – A comprehensive guide to understanding and implementing AI in industrial settings.
- IoT-Enhanced Processors Increase Performance, AI, Security – Details on the latest IoT processors from Intel that enhance performance and security.
- Industrial Cybersecurity Technology for ICS/OT Asset Visibility | Dragos – Learn about technologies for securing industrial control systems and operational technology.
- OT/ICS and Industrial IoT Security – Cisco – Explore Cisco’s solutions for securing industrial networks.
- Protect your extended asset attack surface | Armis – Information on how to protect your assets against cyber threats with Armis.
Disclaimer
This article is AI-generated for educational purposes and does not intend to provide specific advice or recommendations for any individual or on any specific security or investment product. It is only intended to provide education about the financial industry. To discuss your specific situation, please consult a qualified professional.Latest posts by Leo Celis (see all)
- You are in a Reorg Bubble about to Explode - 04/22/25
- The Wrong AI Tool Is As Bad As the Wrong Hire - 04/15/25
- COVID, Remote Work & AI - 04/08/25