Table of Contents
AI-driven predictive analytics is revolutionizing the cybersecurity landscape by enabling organizations to anticipate and mitigate threats before they materialize. This article delves into the key aspects of AI predictive analytics in cybersecurity, the challenges faced by the industry, and practical solutions to enhance security measures.
Understanding AI-Driven Predictive Analytics
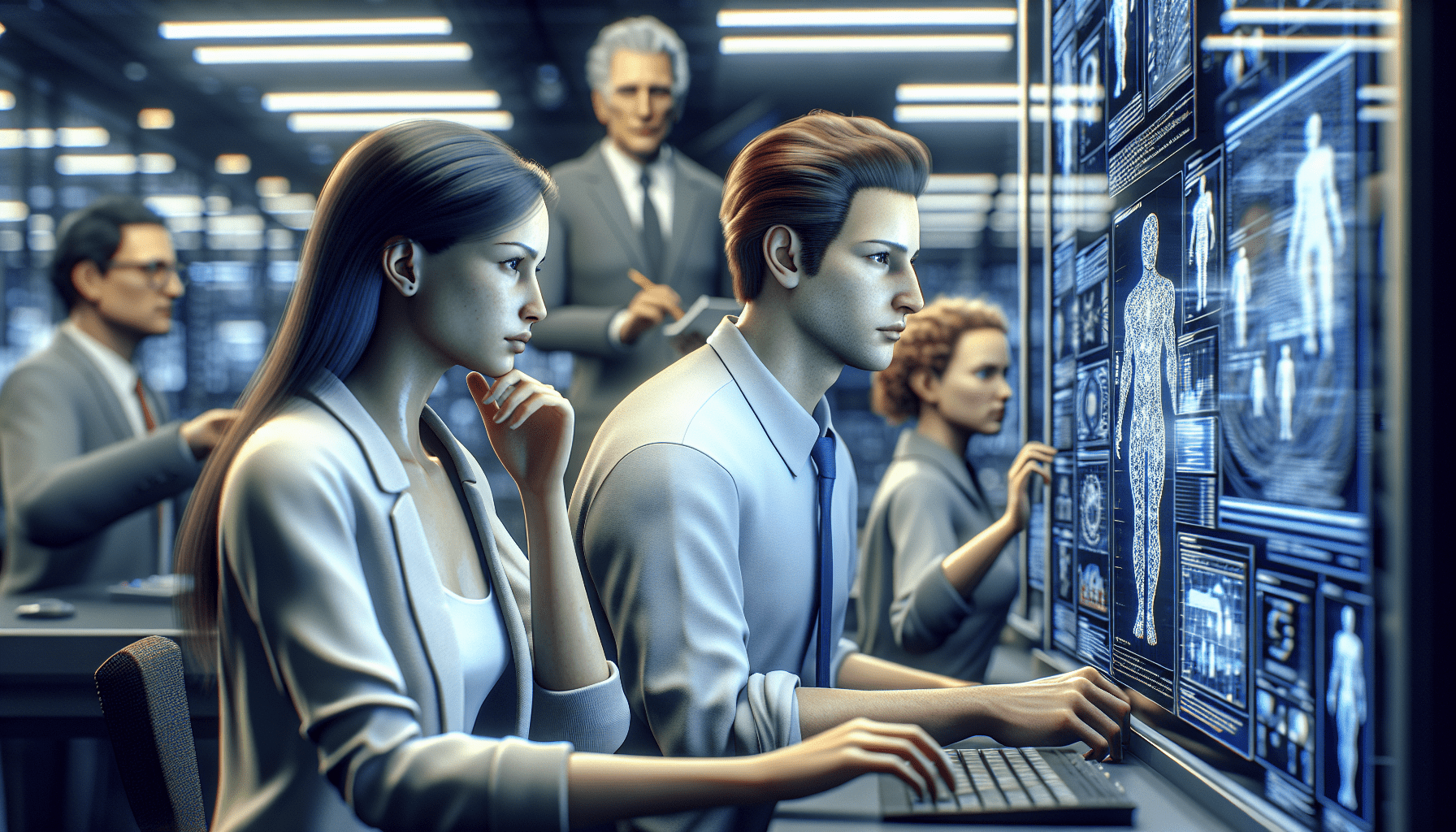
Key Points
- Proactive Threat Detection: AI predictive analytics helps in identifying potential threats before they occur.
- Data-Driven Insights: Leveraging historical data to forecast future cyber threats.
- Automated Response: Enhancing incident response through automation.
- Behavioral Analysis: Monitoring user behavior to detect anomalies.
- Scalability: Handling large volumes of data efficiently.
What is AI-Driven Predictive Analytics?
AI-driven predictive analytics involves using artificial intelligence to analyze historical data and predict future events. In cybersecurity, this means identifying potential threats based on patterns and trends observed in past data. By leveraging machine learning algorithms, AI can forecast the likelihood of various cyber threats, enabling organizations to take preemptive measures.
Predictive analytics in cybersecurity is not just about identifying threats but also about understanding the context in which these threats occur. This involves analyzing user behavior, network traffic, and other relevant data points to create a comprehensive security strategy. The goal is to stay one step ahead of cybercriminals by anticipating their moves and mitigating risks before they can cause harm.
One of the key advantages of AI-driven predictive analytics is its ability to process vast amounts of data quickly and accurately. Traditional methods of threat detection often rely on manual analysis, which can be time-consuming and prone to errors. AI, on the other hand, can analyze data in real-time, providing immediate insights and allowing for faster response times.
How Does AI Predictive Analytics Work?
AI predictive analytics works by using machine learning algorithms to analyze historical data and identify patterns that indicate potential threats. These algorithms are trained on large datasets, which include information about past cyber attacks, user behavior, and network traffic. By identifying patterns and trends in this data, AI can predict the likelihood of future threats and provide actionable insights.
One common approach to predictive analytics in cybersecurity is anomaly detection. This involves monitoring network traffic and user behavior to identify deviations from normal patterns. For example, if a user suddenly starts accessing sensitive data at unusual times, this could indicate a potential security breach. AI can flag these anomalies and alert security teams, allowing them to investigate and take appropriate action.
Another important aspect of AI predictive analytics is its ability to learn and adapt over time. As new data is collected, the algorithms can be retrained to improve their accuracy and effectiveness. This continuous learning process ensures that the AI system remains up-to-date with the latest threats and can provide accurate predictions even as the threat landscape evolves.
Challenges in Cybersecurity
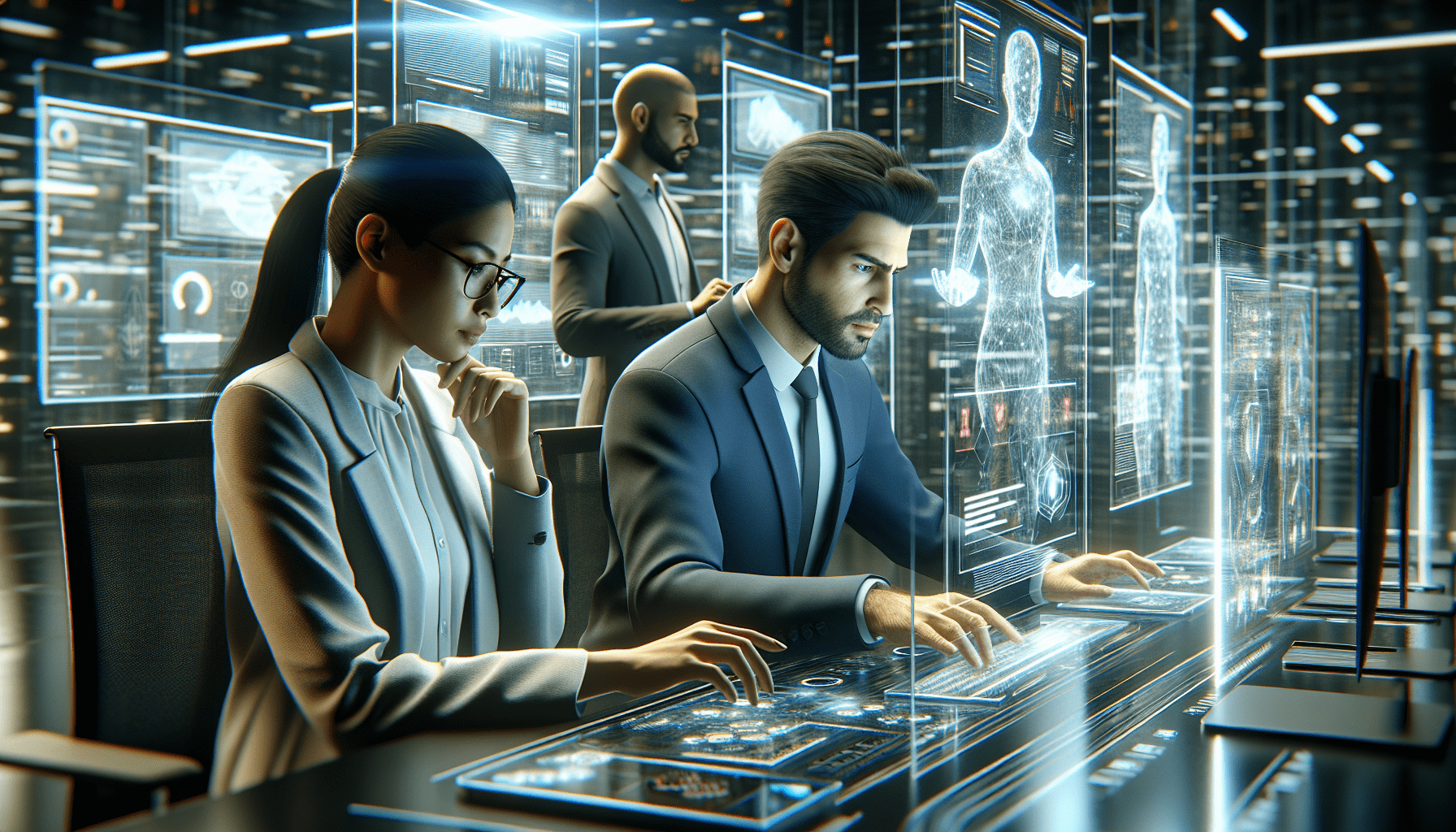
Increasing Sophistication of Cyber Attacks
One of the biggest challenges in cybersecurity today is the increasing sophistication of cyber attacks. Cybercriminals are constantly developing new techniques and strategies to bypass security measures and exploit vulnerabilities. This makes it difficult for organizations to keep up and protect their systems effectively.
Traditional security measures, such as firewalls and antivirus software, are often not enough to defend against these advanced threats. Cybercriminals can use techniques like social engineering, zero-day exploits, and advanced persistent threats (APTs) to infiltrate networks and steal sensitive data. As a result, organizations need more advanced tools and strategies to stay ahead of these evolving threats.
Data Overload
Another major challenge in cybersecurity is the sheer volume of data that needs to be analyzed. Modern organizations generate vast amounts of data every day, including network traffic, user activity logs, and system performance metrics. Analyzing this data manually is not only time-consuming but also prone to errors.
This data overload can make it difficult for security teams to identify potential threats and respond in a timely manner. Without the right tools and technologies, organizations may struggle to process and analyze this data effectively, leaving them vulnerable to cyber attacks.
Resource Constraints
Many organizations also face resource constraints when it comes to cybersecurity. Hiring and retaining skilled cybersecurity professionals can be challenging, especially for smaller organizations with limited budgets. This can make it difficult to implement and maintain effective security measures.
In addition to personnel constraints, organizations may also face budget limitations that prevent them from investing in the latest security technologies. This can leave them at a disadvantage when it comes to defending against advanced threats and staying ahead of cybercriminals.
Implementing AI-Driven Predictive Analytics
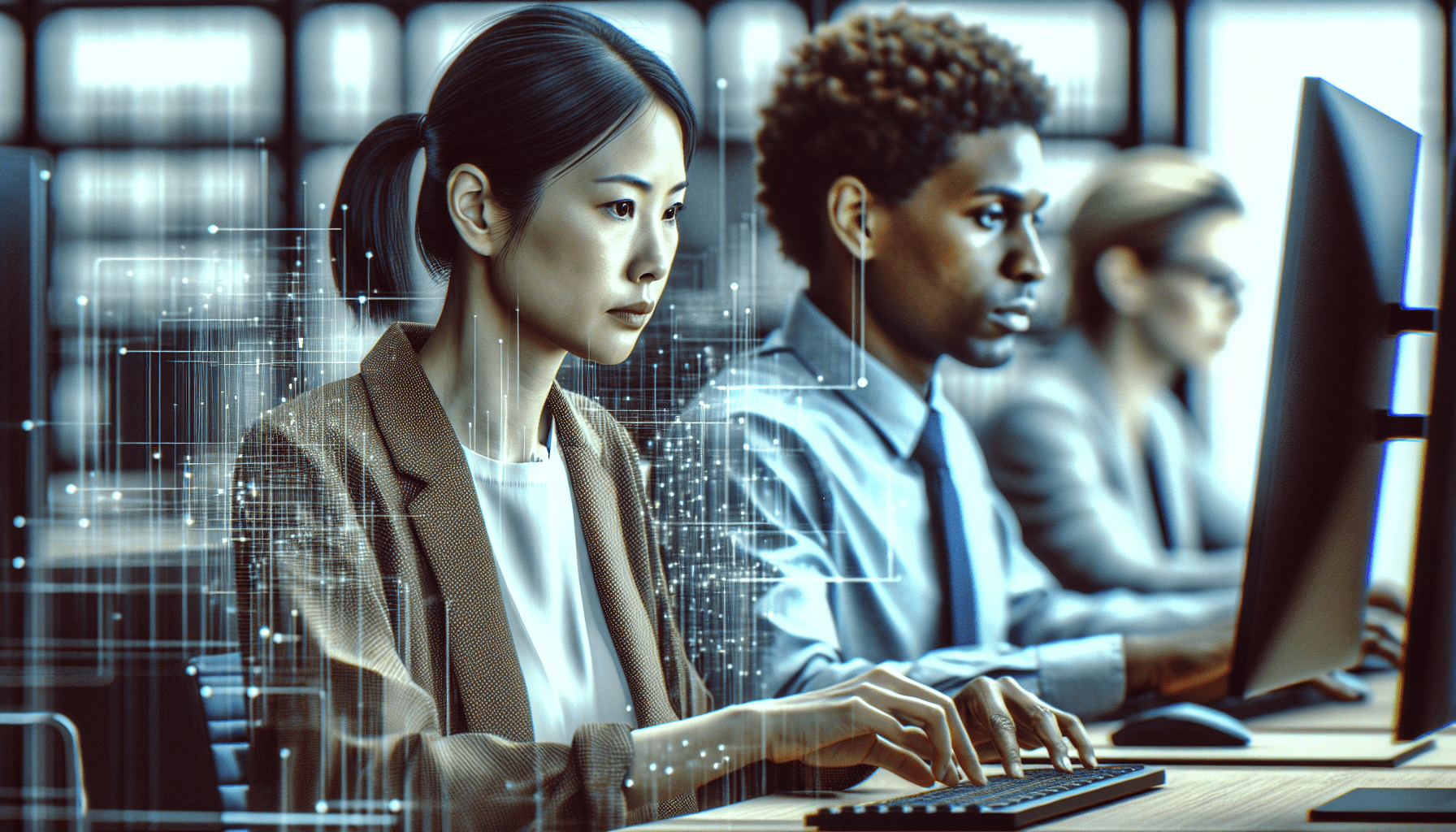
Step 1: Data Collection and Preparation
The first step in implementing AI-driven predictive analytics is to collect and prepare the necessary data. This involves gathering historical data on past cyber attacks, user behavior, and network traffic. The data should be cleaned and preprocessed to ensure it is accurate and relevant for analysis.
Organizations can use various tools and technologies to collect and store this data, such as security information and event management (SIEM) systems, log management tools, and network monitoring solutions. Once the data is collected, it should be organized and labeled to facilitate analysis by machine learning algorithms.
Step 2: Training Machine Learning Models
Once the data is prepared, the next step is to train machine learning models to analyze the data and identify patterns that indicate potential threats. This involves selecting the appropriate algorithms and training them on the historical data. The models should be evaluated and fine-tuned to ensure they provide accurate and reliable predictions.
Organizations can use various machine learning techniques for predictive analytics, such as supervised learning, unsupervised learning, and reinforcement learning. The choice of technique will depend on the specific use case and the nature of the data being analyzed. It is important to continuously monitor and update the models to ensure they remain effective as new data is collected.
Step 3: Implementing Predictive Analytics Solutions
The final step is to implement the predictive analytics solutions and integrate them into the organization’s existing security infrastructure. This involves deploying the trained models and setting up automated processes to monitor network traffic and user behavior in real-time.
Organizations should also establish protocols for responding to potential threats identified by the predictive analytics system. This may include setting up alerts, automating incident response actions, and conducting regular security assessments to ensure the system is functioning effectively.
FAQs
What is AI-driven predictive analytics in cybersecurity?
AI-driven predictive analytics in cybersecurity involves using artificial intelligence to analyze historical data and predict future cyber threats. This helps organizations identify potential threats before they occur and take preemptive measures to mitigate risks.
How does AI improve threat detection?
AI improves threat detection by using machine learning algorithms to analyze large volumes of data and identify patterns that indicate potential threats. This allows for real-time monitoring and faster response times, reducing the risk of security breaches.
What are the benefits of using AI in cybersecurity?
The benefits of using AI in cybersecurity include proactive threat detection, data-driven insights, automated response, behavioral analysis, and scalability. AI can process vast amounts of data quickly and accurately, providing immediate insights and enhancing overall security measures.
What challenges do organizations face in implementing AI-driven predictive analytics?
Organizations face challenges such as the increasing sophistication of cyber attacks, data overload, and resource constraints. These challenges can make it difficult to implement and maintain effective security measures, highlighting the need for advanced tools and technologies like AI-driven predictive analytics.
Future of AI-Driven Predictive Analytics in Cybersecurity
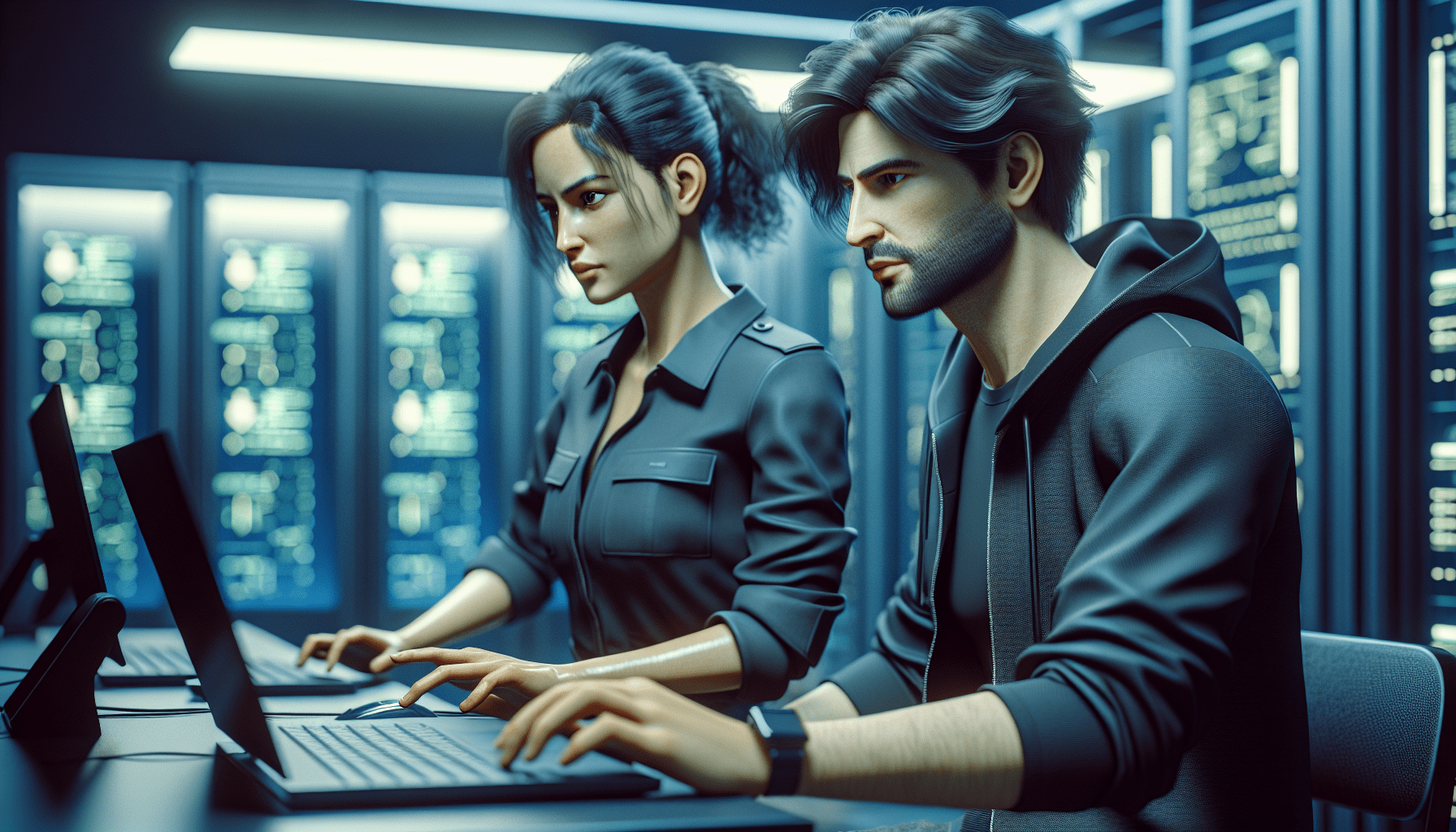
The future of AI-driven predictive analytics in cybersecurity looks promising, with several key trends and developments expected to shape the industry. Here are five predictions for the future:
- Increased Adoption of AI: More organizations will adopt AI-driven predictive analytics to enhance their cybersecurity measures and stay ahead of evolving threats.
- Advancements in Machine Learning: Continued advancements in machine learning algorithms will improve the accuracy and effectiveness of predictive analytics in identifying potential threats.
- Integration with Other Technologies: AI-driven predictive analytics will be integrated with other emerging technologies, such as blockchain and IoT, to provide more comprehensive security solutions.
- Focus on User Behavior Analysis: There will be a greater emphasis on analyzing user behavior to detect anomalies and potential security breaches.
- Enhanced Automation: Automation will play a key role in incident response, with AI-driven systems automatically taking action to mitigate threats and reduce response times.
More Information
- How Predictive Analytics Could Change Cybersecurity – An article discussing the impact of predictive analytics on cybersecurity.
- What is Predictive AI & How is It Used in Cybersecurity? – A blog post explaining the concept of predictive AI and its applications in cybersecurity.
- New introductory courses in gen AI, data analytics & cybersecurity | Google Cloud Blog – Information about new courses in AI, data analytics, and cybersecurity offered by Google Cloud.
Disclaimer
This is an AI-generated article with educative purposes and doesn’t intend to give advice or recommend its implementation. The goal is to inspire readers to research and delve deeper into the topics covered in the article.
- The AI Automation Engineer - 05/13/25
- Hire One Developer to Press One Key - 05/06/25
- Stop Consuming AI and Start Riding AI - 04/29/25