Table of Contents
AI bias in advertising is a significant concern in the ad-tech industry.
This article explores how to mitigate bias in AI-driven advertising, ensuring fair and inclusive marketing practices.
Understanding AI Bias in Advertising
Key Points
- AI bias can perpetuate stereotypes and exclusionary practices.
- Diverse training data is crucial for reducing bias.
- Regular monitoring and auditing of AI models are essential.
- Human oversight helps ensure ethical standards.
- Prompt engineering can improve AI inclusivity.
Definition and Causes
AI bias in advertising occurs when AI algorithms produce results that are systematically prejudiced due to biased training data. This bias can stem from historical data that reflects societal prejudices, leading to discriminatory or exclusionary marketing content. For instance, an AI model might use masculine pronouns by default, reinforcing gender stereotypes.
Bias can also arise from the lack of diversity in training data. If the data used to train AI models predominantly represents one demographic, the resulting AI outputs may not be inclusive. This can lead to marketing content that alienates certain groups of consumers.
Moreover, the feedback loop in AI models can perpetuate bias. If biased responses are not appropriately monitored and addressed, the AI model can learn and reinforce these biases over time, creating a cycle of biased content and feedback.
Impact on Advertising
The impact of AI bias in advertising is significant. Biased AI models can generate marketing content that reinforces stereotypes and excludes certain demographics. For example, an AI model might generate images of men in leadership roles and women in customer-facing roles, perpetuating gender biases.
Additionally, biased AI models can target marketing content based on gender, displaying ads for cars and bikes to men and ads for cosmetics to women. This not only reinforces stereotypes but also limits the reach of marketing campaigns to a broader audience.
Furthermore, the lack of diversity in training data can lead to biased audience segmentation. If the training data is limited in terms of representation across various demographic groups, the resulting AI model may exhibit biased marketing content, affecting the overall effectiveness of advertising campaigns.
Examples of Bias
Several examples illustrate the presence of AI bias in advertising. One common example is the generation of images or videos that depict only one gender in certain roles. AI models might provide images of men in construction-related field roles and images of women in hospitality or healthcare-related roles.
Another example is the targeting of marketing content based on gender. The algorithm might display ads for cars and bikes to men and ads for cosmetics to women. This reinforces gender stereotypes and limits the reach of marketing campaigns.
Lastly, the lack of diversity in training data can lead to biased audience segmentation. If the training data is limited in terms of representation across various demographic groups, the resulting AI model may exhibit biased marketing content, affecting the overall effectiveness of advertising campaigns.
The Challenge of Bias in AI-Driven Advertising
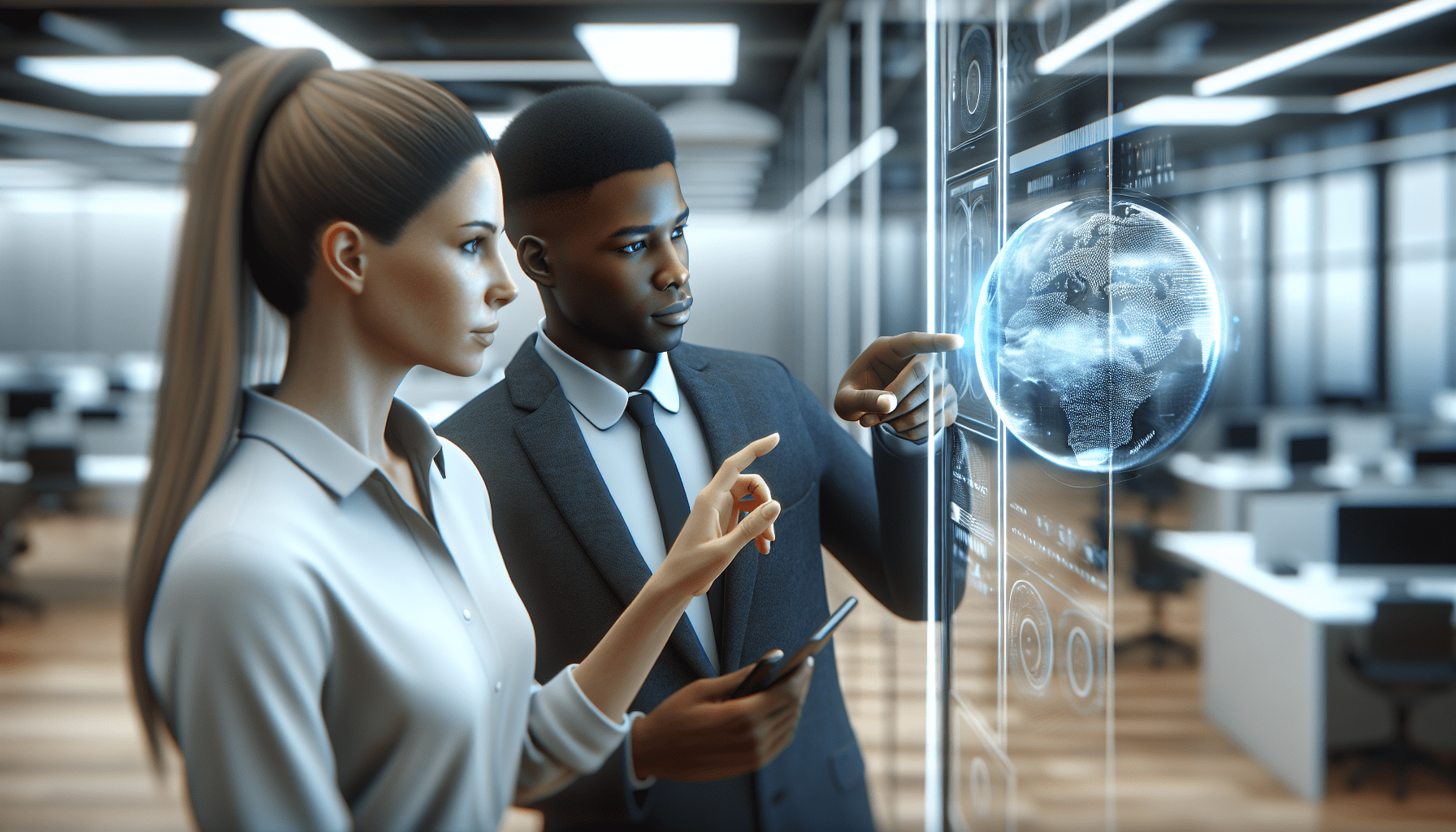
Identifying the Problem
AI bias in advertising is a significant challenge in the ad-tech industry. One of the most challenging problems is the perpetuation of gender stereotypes in marketing content. AI models trained on biased data can generate content that reinforces these stereotypes, leading to discriminatory or exclusionary messaging.
For example, an AI model might generate images of men in leadership roles and women in customer-facing roles, perpetuating the bias that women are not capable of holding leadership positions. This not only reinforces stereotypes but also limits the reach of marketing campaigns to a broader audience.
Impact on Ad Performance
The impact of AI bias in advertising on ad performance is significant. Biased AI models can generate marketing content that alienates certain groups of consumers, leading to lower engagement and conversion rates. For instance, if an AI model generates content that reinforces gender stereotypes, it may alienate female consumers, leading to lower engagement and conversion rates.
Moreover, biased AI models can limit the reach of marketing campaigns. If the training data is limited in terms of representation across various demographic groups, the resulting AI model may exhibit biased marketing content, affecting the overall effectiveness of advertising campaigns.
Ethical Considerations
Ethical considerations are crucial when addressing AI bias in advertising. It is essential to ensure that AI models are trained on diverse and representative data to reduce bias. Additionally, regular monitoring and auditing of AI models are necessary to identify and address any biases that may arise.
Human oversight is also essential to ensure that the content generated by AI models aligns with ethical and inclusive standards. By involving human oversight, marketers can ensure that the content generated by AI models is fair and inclusive, reducing the risk of discriminatory or exclusionary messaging.
Steps to Mitigate AI Bias in Advertising
Step 1: Invest in Diverse Training Data
Investing in diverse training data is crucial for reducing AI bias in advertising. By ensuring that the training data represents various demographic groups, cultural backgrounds, and regions, marketers can develop AI models that generate fair and inclusive marketing content.
For example, including images of both men and women in leadership roles in the training data can help reduce gender bias in the content generated by AI models. Additionally, incorporating diverse cultural references and slang can help AI models better understand and generate content that resonates with a broader audience.
Step 2: Regular Monitoring and Auditing
Regular monitoring and auditing of AI models are essential to identify and address any biases that may arise. By regularly reviewing the content generated by AI models, marketers can identify any biases and take corrective action to ensure that the content is fair and inclusive.
For instance, if an AI model generates content that reinforces gender stereotypes, marketers can take corrective action by retraining the model with more diverse and representative data. Additionally, regular audits can help identify any biases that may arise over time, ensuring that the AI models continue to generate fair and inclusive content.
Step 3: Involve Human Oversight
Involving human oversight is crucial to ensure that the content generated by AI models aligns with ethical and inclusive standards. By involving human oversight, marketers can ensure that the content generated by AI models is fair and inclusive, reducing the risk of discriminatory or exclusionary messaging.
For example, marketers can review the content generated by AI models to ensure that it does not reinforce stereotypes or exclude certain groups of consumers. Additionally, human oversight can help ensure that the content generated by AI models aligns with the brand’s values and messaging, ensuring that the marketing content is both effective and ethical.
FAQs
What is AI bias in advertising?
AI bias in advertising occurs when AI algorithms produce results that are systematically prejudiced due to biased training data. This can lead to discriminatory or exclusionary marketing content.
How can AI bias in advertising be reduced?
AI bias in advertising can be reduced by investing in diverse training data, regularly monitoring and auditing AI models, and involving human oversight to ensure that the content generated by AI models is fair and inclusive.
Why is diverse training data important?
Diverse training data is important because it ensures that AI models represent various demographic groups, cultural backgrounds, and regions. This helps develop AI models that generate fair and inclusive marketing content.
What role does human oversight play in mitigating AI bias?
Human oversight is crucial in mitigating AI bias as it ensures that the content generated by AI models aligns with ethical and inclusive standards. It helps reduce the risk of discriminatory or exclusionary messaging.
Future of AI Bias Mitigation in Advertising
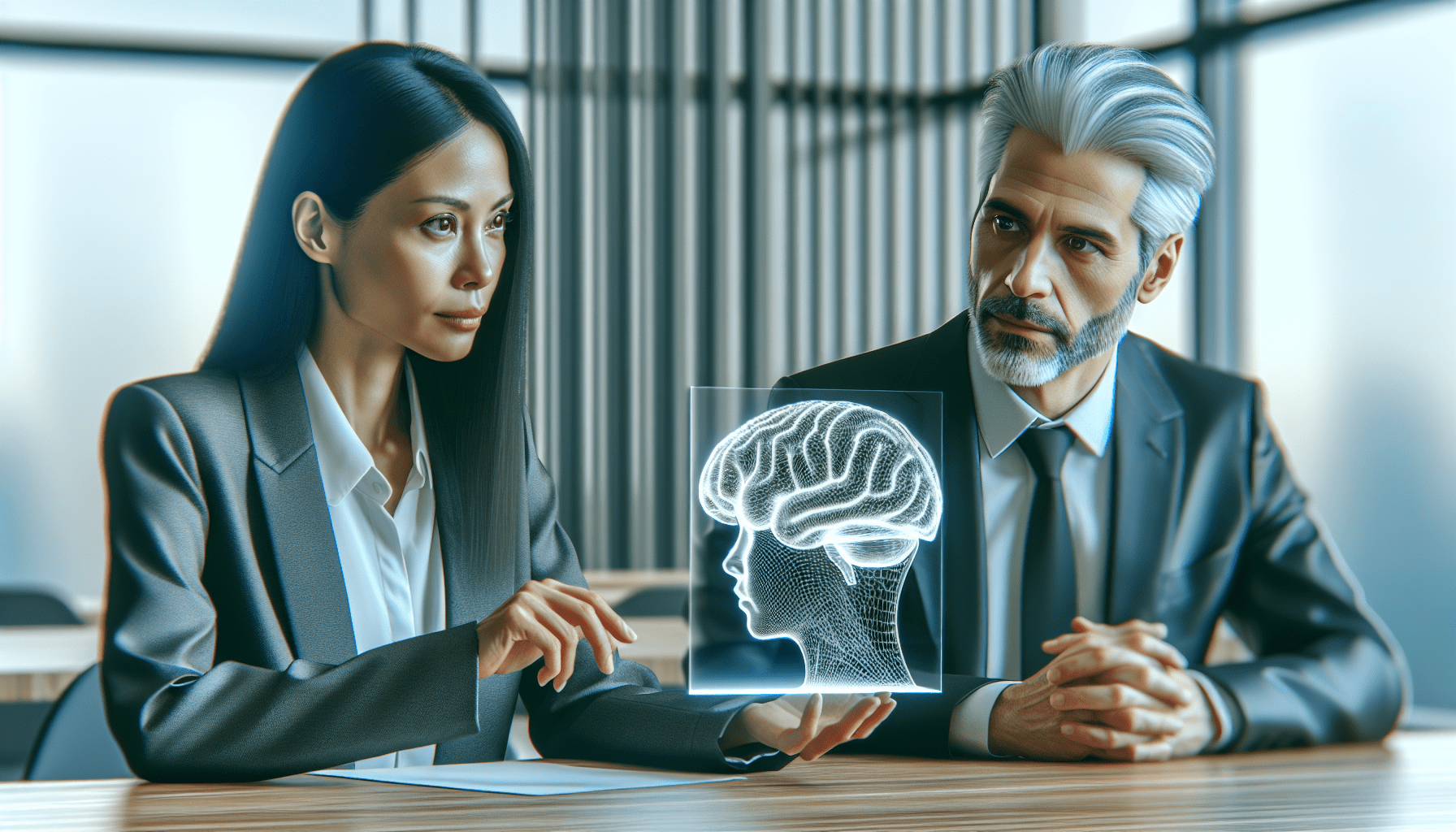
The future of AI bias in advertising is evolving, with several trends and predictions shaping the landscape. Here are five robust predictions backed by real trend data:
- Increased investment in diverse training data: Companies will invest more in diverse and representative training data to reduce AI bias.
- Advancements in AI auditing tools: New tools and technologies will emerge to help marketers regularly monitor and audit AI models for bias.
- Greater emphasis on ethical AI practices: Ethical considerations will become a central focus in AI development, ensuring fair and inclusive marketing content.
- Collaboration between AI developers and marketers: Increased collaboration between AI developers and marketers will help create more inclusive AI models.
- Regulatory frameworks for AI bias: Governments and regulatory bodies will develop frameworks to address AI bias, ensuring fair and inclusive advertising practices.
More Information
- Overcoming Algorithmic Gender Bias In AI-Generated Marketing Content – An article discussing the challenges and solutions for gender bias in AI-generated marketing content.
- A French ad campaign centered on AI bias (and a movement to “correct” AI data?) – An analysis of a French ad campaign addressing AI bias and efforts to correct AI data.
- Bloomberg – Are you a robot? – A newsletter discussing how major companies are using AI to avoid bias and clichés in advertising.
Disclaimer
This is an AI-generated article with educative purposes and doesn’t intend to give advice or recommend its implementation. The goal is to inspire readers to research and delve deeper into the topics covered in the article.
- From SaaS to AI Agents - 05/27/25
- The AI Automation Engineer - 05/13/25
- Hire One Developer to Press One Key - 05/06/25