Table of Contents
Introduction
In the rapidly evolving world of software development, the integration of artificial intelligence (AI) has become a cornerstone for innovation. However, with this advancement comes the challenge of ensuring fairness and equity in AI-driven systems. The concept of “Human-in-the-Loop” for bias mitigation is gaining traction as a vital strategy to address these concerns. By incorporating human oversight into AI processes, companies can significantly reduce biases, leading to more ethical and effective outcomes.
For marketing managers, digital strategists, and executives in the software development industry, understanding how to implement human-in-the-loop systems is crucial. This approach not only enhances the reliability of AI systems but also aligns with the growing demand for ethical AI practices. As businesses increasingly rely on AI for decision-making, the need for bias mitigation becomes paramount to maintain trust and integrity in AI applications.
Comprehensive Overview of Human-in-the-Loop for Bias Mitigation
Key Points
- Human-in-the-loop systems integrate human judgment to reduce AI bias.
- These systems are essential for ethical AI deployment in software development.
- Implementing human oversight can improve AI-driven advertising strategies.
- Understanding bias mitigation is crucial for enhancing ROI in AI applications.
Understanding Human-in-the-Loop Systems
Human-in-the-loop (HITL) systems are designed to incorporate human judgment into AI processes, ensuring that decisions made by AI are reviewed and adjusted by humans. This approach is particularly important in areas where AI systems are prone to biases, such as in advertising and marketing strategies. By involving humans in the loop, companies can ensure that AI-driven decisions are fair and equitable.
HITL systems work by allowing humans to intervene at critical points in the AI decision-making process. This intervention can occur during data collection, model training, or even after the AI has made a decision. The goal is to identify and correct biases that may have been introduced at any stage of the AI lifecycle.
One key benefit of HITL systems is their ability to adapt to new information and changing circumstances. Unlike fully automated systems, HITL systems can incorporate human insights and expertise, making them more flexible and responsive to real-world challenges.
Implementing HITL systems can lead to more reliable and trustworthy AI applications for software development companies. By reducing biases, these systems can improve the effectiveness of AI-driven advertising strategies, ultimately enhancing ROI.
The Role of Human Oversight in AI
Human oversight plays a critical role in ensuring the ethical deployment of AI systems. In the context of bias mitigation, human oversight involves monitoring AI processes to identify and address potential biases. This oversight can take various forms, including regular audits, feedback loops, and continuous monitoring of AI outputs.
One of the primary challenges in AI bias mitigation is identifying biases that may not be immediately apparent. Human oversight can help uncover these hidden biases by providing a fresh perspective and critical analysis of AI decisions. This is particularly important in industries where AI is used for high-stakes decision-making, such as finance, healthcare, and advertising.
Human oversight can identify biases and help develop strategies for mitigating them. This may involve adjusting AI models, refining data inputs, or implementing new policies and procedures to ensure fairness and equity in AI applications.
For companies looking to enhance their AI-driven advertising strategies, human oversight is an essential component of bias mitigation. By ensuring that AI systems are fair and unbiased, companies can build trust with their customers and stakeholders, ultimately leading to better business outcomes.
Industry-Specific Challenges in Bias Mitigation
Challenge 1: Data Bias in AI Systems
Data bias is a significant challenge in the software development industry, particularly when it comes to AI applications. Biases in data can arise from various sources, including historical inequalities, unrepresentative datasets, and flawed data collection methods. These biases can lead to unfair and discriminatory outcomes in AI-driven systems.
For companies relying on AI for advertising strategies, data bias can result in targeting inaccuracies and reduced effectiveness. This can ultimately impact ROI and customer satisfaction. Addressing data bias requires a comprehensive approach that includes data auditing, cleansing, and validation to ensure that datasets are representative and free from bias.
One effective strategy for mitigating data bias is to involve diverse teams in the data collection and analysis process. By incorporating diverse perspectives, companies can identify potential biases and develop strategies to address them. This approach not only improves the quality of the data but also enhances the overall effectiveness of AI systems.
For marketing managers and digital strategists, understanding the sources of data bias and implementing strategies to mitigate it is crucial for developing effective AI-driven advertising strategies. By addressing data bias, companies can improve the accuracy and fairness of their AI applications, leading to better business outcomes.
Challenge 2: Algorithmic Bias in AI Models
Algorithmic bias is another significant challenge in the software development industry. This type of bias occurs when AI models produce biased outcomes due to flaws in their design or training. Algorithmic bias can result in unfair and discriminatory decisions, impacting the effectiveness and reliability of AI systems.
For companies using AI for advertising strategies, algorithmic bias can lead to targeting inaccuracies and reduced effectiveness. This can ultimately impact ROI and customer satisfaction. Addressing algorithmic bias requires a comprehensive approach that includes model auditing, testing, and validation to ensure that AI models are fair and unbiased.
One effective strategy for mitigating algorithmic bias is to involve diverse teams in the model development and testing process. By incorporating diverse perspectives, companies can identify potential biases and develop strategies to address them. This approach not only improves the quality of the AI models but also enhances the overall effectiveness of AI systems.
For marketing managers and digital strategists, understanding the sources of algorithmic bias and implementing strategies to mitigate it is crucial for developing effective AI-driven advertising strategies. By addressing algorithmic bias, companies can improve the accuracy and fairness of their AI applications, leading to better business outcomes.
Challenge 3: Lack of Transparency in AI Systems
Lack of transparency is a significant challenge in the software development industry, particularly when it comes to AI applications. Transparency is essential for building trust and ensuring the ethical deployment of AI systems. Without transparency, it can be difficult to identify and address biases in AI-driven systems.
Lack of transparency can result in targeting inaccuracies and reduced effectiveness for companies relying on AI for advertising strategies. This can ultimately impact ROI and customer satisfaction. Addressing transparency requires a comprehensive approach that includes clear communication, documentation, and reporting of AI processes and decisions.
One effective strategy for improving transparency is to involve diverse teams in the development and deployment of AI systems. By incorporating diverse perspectives, companies can ensure that AI processes are transparent and understandable to all stakeholders. This approach not only improves the quality of the AI systems but also enhances the overall effectiveness of AI applications.
For marketing managers and digital strategists, understanding the importance of transparency and implementing strategies to improve it is crucial for developing effective AI-driven advertising strategies. By improving transparency, companies can build trust with their customers and stakeholders, ultimately leading to better business outcomes.
Challenge 4: Ethical Considerations in AI Deployment
Ethical considerations are a significant challenge in the software development industry, particularly when it comes to AI applications. Ensuring the ethical deployment of AI systems is essential for building trust and maintaining the integrity of AI-driven systems. Without ethical considerations, AI systems can produce biased and discriminatory outcomes.
For companies relying on AI for advertising strategies, ethical considerations can impact targeting accuracy and effectiveness. This can ultimately impact ROI and customer satisfaction. Addressing ethical considerations requires a comprehensive approach that includes ethical guidelines, policies, and procedures for AI deployment.
One effective strategy for addressing ethical considerations is to involve diverse teams in the development and deployment of AI systems. By incorporating diverse perspectives, companies can ensure that AI systems are ethical and fair. This approach not only improves the quality of the AI systems but also enhances the overall effectiveness of AI applications.
For marketing managers and digital strategists, understanding the importance of ethical considerations and implementing strategies to address them is crucial for developing effective AI-driven advertising strategies. By addressing ethical considerations, companies can build trust with their customers and stakeholders, ultimately leading to better business outcomes.
Step-by-Step Solutions for Bias Mitigation
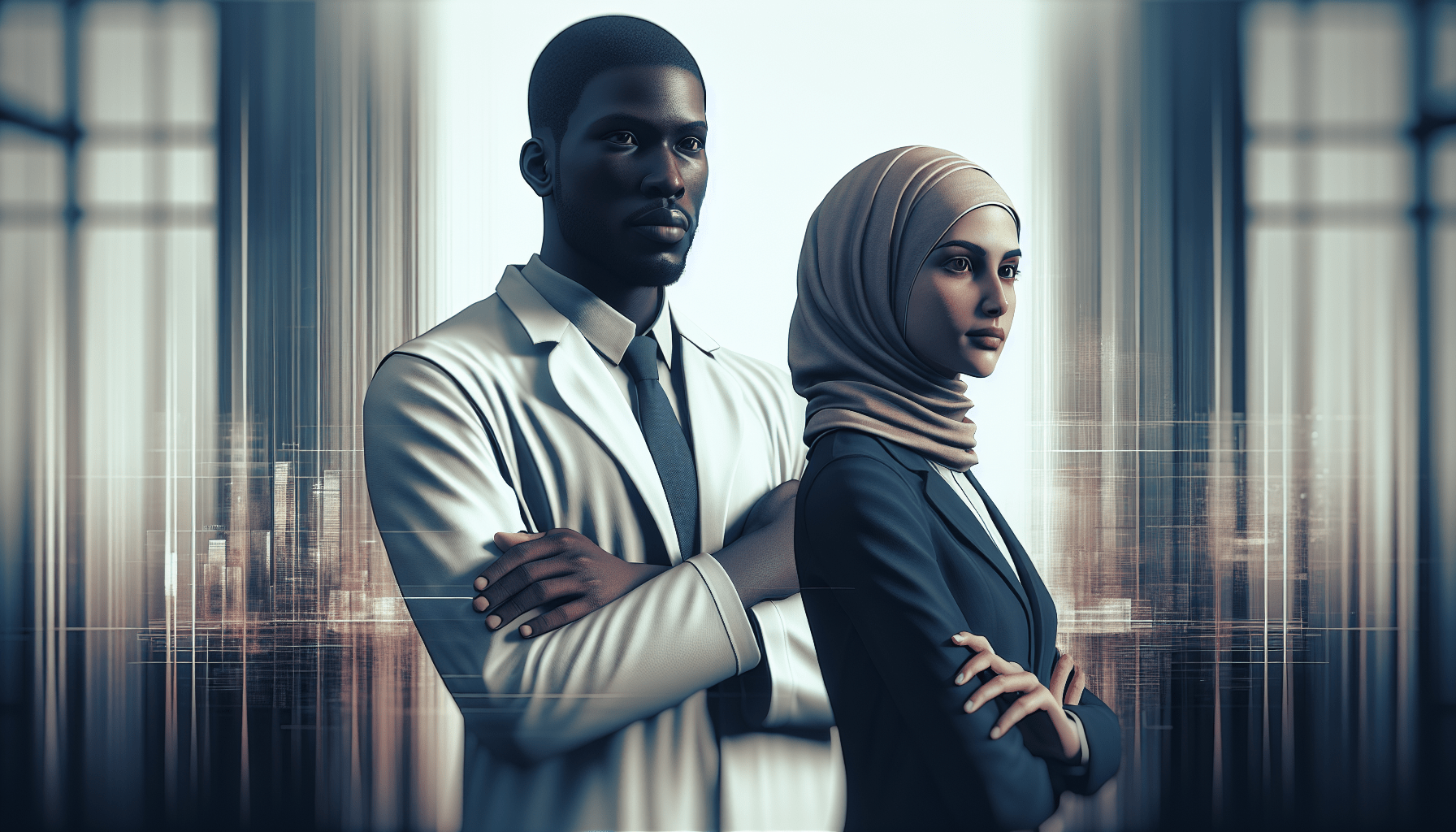
Step 1: Implementing Human-in-the-Loop Systems
Implementing human-in-the-loop systems is a crucial step in bias mitigation. These systems involve human oversight at critical points in the AI decision-making process, ensuring that biases are identified and addressed. By incorporating human judgment, companies can improve the fairness and accuracy of AI-driven systems.
To implement human-in-the-loop systems, companies should first identify the key decision points in their AI processes where human intervention is needed. This may include data collection, model training, or decision-making stages. By pinpointing these areas, companies can ensure that human oversight is effectively integrated into their AI systems.
Next, companies should establish clear guidelines and procedures for human intervention. This includes defining the roles and responsibilities of human reviewers, as well as the criteria for identifying and addressing biases. By setting clear expectations, companies can ensure that human oversight is consistent and effective.
Finally, companies should continuously monitor and evaluate the effectiveness of their human-in-the-loop systems. This involves regularly reviewing AI decisions and outcomes to identify any biases that may have been missed. By maintaining a proactive approach, companies can ensure that their AI systems remain fair and unbiased.
Step 2: Enhancing Data Quality and Diversity
Enhancing data quality and diversity is another critical step in bias mitigation. Data biases can significantly impact the fairness and accuracy of AI-driven systems, making it essential to ensure that datasets are representative and free from bias.
To enhance data quality and diversity, companies should first conduct a thorough audit of their existing datasets. This involves identifying any biases or gaps in the data and developing strategies to address them. By understanding their data’s limitations, companies can take steps to improve its quality and representativeness.
Next, companies should actively seek out diverse data sources to supplement their existing datasets. This may involve collaborating with external partners or leveraging publicly available data to ensure a more comprehensive and representative dataset. By incorporating diverse data sources, companies can improve the accuracy and fairness of their AI systems.
Finally, companies should implement ongoing data validation and cleansing processes to ensure that their datasets remain free from bias. This involves regularly reviewing and updating data inputs to address any changes or new information. By maintaining high data quality, companies can ensure that their AI systems remain fair and effective.
Step 3: Developing Transparent AI Processes
Developing transparent AI processes is essential for building trust and ensuring the ethical deployment of AI systems. Transparency allows stakeholders to understand how AI decisions are made and identify any potential biases or issues.
To develop transparent AI processes, companies should first establish clear documentation and reporting procedures for their AI systems. This includes detailing the data inputs, model parameters, and decision-making criteria used in AI processes. By providing comprehensive documentation, companies can ensure that their AI systems are transparent and understandable to all stakeholders.
Next, companies should implement regular audits and reviews of their AI processes to identify any biases or issues. This involves involving diverse teams in the review process to ensure a comprehensive and unbiased analysis. By regularly reviewing AI processes, companies can identify and address any potential biases or issues.
Finally, companies should communicate their AI processes and decisions to stakeholders clearly and accessiblely. This includes providing regular updates and reports on AI decisions and outcomes and addressing any concerns or questions from stakeholders. By maintaining open communication, companies can build trust and ensure the ethical deployment of their AI systems.
Step 4: Establishing Ethical Guidelines for AI Deployment
Establishing ethical guidelines for AI deployment is crucial for ensuring the fairness and integrity of AI-driven systems. Ethical guidelines provide a framework for making decisions and addressing biases in AI processes.
To establish ethical guidelines, companies should first identify the key ethical considerations relevant to their AI systems. This may include issues related to fairness, transparency, privacy, and accountability. By understanding the ethical implications of their AI systems, companies can develop guidelines that address these concerns.
Next, companies should involve diverse teams in the development of ethical guidelines to ensure a comprehensive and inclusive approach. This involves incorporating diverse perspectives and expertise to identify potential biases and develop strategies to address them. By involving diverse teams, companies can ensure that their ethical guidelines are fair and representative.
Finally, companies should regularly review and update their ethical guidelines to ensure that they remain relevant and effective. This involves monitoring changes in technology, regulations, and societal expectations to ensure that ethical guidelines continue to address emerging challenges. By maintaining up-to-date ethical guidelines, companies can ensure the fairness and integrity of their AI systems.
Code Example for Bias Mitigation
In this section, we will provide a code example that demonstrates how to implement a human-in-the-loop system for bias mitigation in AI models. The code will include classes and methods to facilitate human oversight and intervention in AI decision-making processes.
class HumanInTheLoop:
def __init__(self, model, data):
"""
Initialize the HumanInTheLoop system with a model and data.
:param model: The AI model to be monitored.
:param data: The dataset used for training and evaluation.
"""
self.model = model
self.data = data
self.human_reviewers = []
def add_human_reviewer(self, reviewer):
"""
Add a human reviewer to the system.
:param reviewer: A function or callable that performs human review.
"""
self.human_reviewers.append(reviewer)
def evaluate(self):
"""
Evaluate the model with human oversight.
:return: A report of the evaluation results.
"""
results = self.model.predict(self.data)
for reviewer in self.human_reviewers:
results = reviewer(results)
return self.generate_report(results)
def generate_report(self, results):
"""
Generate a report based on the evaluation results.
:param results: The results after human review.
:return: A formatted report.
"""
# Example report generation logic
report = f"Evaluation Report:\nBias Detected: {self.detect_bias(results)}\n"
return report
def detect_bias(self, results):
"""
Detect bias in the evaluation results.
:param results: The results to be analyzed.
:return: Boolean indicating if bias was detected.
"""
# Example bias detection logic
return any(result < 0 for result in results)
Code language: Python (python)
This code example demonstrates a simple implementation of a human-in-the-loop system for bias mitigation. The HumanInTheLoop
class is initialized with an AI model and dataset. Human reviewers can be added to the system to provide oversight and review AI decisions. The evaluate
method runs the model’s predictions through the human reviewers, and a report is generated to highlight any detected biases. This approach allows for human intervention at critical points in the AI decision-making process, ensuring that biases are identified and addressed.
FAQs
What is human-in-the-loop in AI?
Human-in-the-loop (HITL) in AI refers to the integration of human judgment and oversight into AI processes. This approach involves human intervention at critical points in the AI decision-making process to ensure that decisions are fair, accurate, and free from bias. HITL systems are particularly important in areas where AI systems are prone to biases, such as in advertising and marketing strategies. By involving humans in the loop, companies can ensure that AI-driven decisions are ethical and equitable.
HITL systems work by allowing humans to intervene during data collection, model training, or even after the AI has made a decision. This intervention helps identify and correct biases that may have been introduced at any stage of the AI lifecycle. The goal is to improve the fairness and accuracy of AI systems by incorporating human insights and expertise.
Implementing HITL systems can lead to more reliable and trustworthy AI applications for software development companies. By reducing biases, these systems can improve the effectiveness of AI-driven advertising strategies, ultimately enhancing ROI. Understanding how to implement HITL systems is crucial for marketing managers, digital strategists, and executives looking to develop ethical AI practices.
Overall, human-in-the-loop systems are a vital strategy for bias mitigation in AI. By incorporating human oversight, companies can ensure that their AI systems are fair, ethical, and effective, leading to better business outcomes and increased trust with customers and stakeholders.
Why is bias mitigation important in AI?
Bias mitigation is crucial in AI because biases in AI systems can lead to unfair and discriminatory outcomes. These biases can arise from various sources, including data biases, algorithmic biases, and lack of transparency. If left unaddressed, biases in AI systems can impact the effectiveness and reliability of AI applications, leading to negative consequences for businesses and individuals.
For companies relying on AI for decision-making, bias mitigation is essential for maintaining trust and integrity in AI applications. By ensuring that AI systems are fair and unbiased, companies can build trust with their customers and stakeholders, ultimately leading to better business outcomes. Bias mitigation is particularly important in industries where AI is used for high-stakes decision-making, such as finance, healthcare, and advertising.
Bias mitigation also aligns with the growing demand for ethical AI practices. As AI systems become more prevalent, companies are expected to ensure that their AI applications are fair and equitable. By addressing biases, companies can demonstrate their commitment to ethical AI practices and build a positive reputation in the industry.
Overall, bias mitigation is a critical component of ethical AI deployment. By addressing biases in AI systems, companies can improve the fairness and accuracy of their AI applications, leading to better business outcomes and increased trust with customers and stakeholders.
How can companies implement human-in-the-loop systems?
Implementing human-in-the-loop (HITL) systems involves integrating human oversight into AI processes to ensure that decisions are fair, accurate, and free from bias. To implement HITL systems, companies should first identify the key decision points in their AI processes where human intervention is needed. This may include data collection, model training, or decision-making stages.
Once the key decision points are identified, companies should establish clear guidelines and procedures for human intervention. This includes defining the roles and responsibilities of human reviewers, as well as the criteria for identifying and addressing biases. By setting clear expectations, companies can ensure that human oversight is consistent and effective.
Next, companies should continuously monitor and evaluate the effectiveness of their HITL systems. This involves regularly reviewing AI decisions and outcomes to identify any biases that may have been missed. By maintaining a proactive approach, companies can ensure that their AI systems remain fair and unbiased.
Finally, companies should involve diverse teams in the development and implementation of HITL systems. By incorporating diverse perspectives, companies can identify potential biases and develop strategies to address them. This approach not only improves the quality of the AI systems but also enhances the overall effectiveness of AI applications.
What are the challenges of bias mitigation in AI?
Bias mitigation in AI presents several challenges, including data bias, algorithmic bias, lack of transparency, and ethical considerations. Data bias occurs when datasets used to train AI models are unrepresentative or flawed, leading to biased outcomes. Addressing data bias requires comprehensive data auditing, cleansing, and validation to ensure that datasets are representative and free from bias.
Algorithmic bias arises from flaws in the design or training of AI models, resulting in biased outcomes. Mitigating algorithmic bias involves model auditing, testing, and validation to ensure that AI models are fair and unbiased. Involving diverse teams in the model development and testing process can help identify potential biases and develop strategies to address them.
Lack of transparency is another challenge in bias mitigation. Transparency is essential for building trust and ensuring the ethical deployment of AI systems. Developing transparent AI processes involves clear communication, documentation, and reporting of AI processes and decisions. Regular audits and reviews can help identify and address any biases or issues.
Ethical considerations are also a significant challenge in bias mitigation. Ensuring the ethical deployment of AI systems requires ethical guidelines, policies, and procedures. Involving diverse teams in the development of ethical guidelines can ensure a comprehensive and inclusive approach. Regularly reviewing and updating ethical guidelines can help address emerging challenges and ensure the fairness and integrity of AI systems.
Future Trends and Predictions in Bias Mitigation
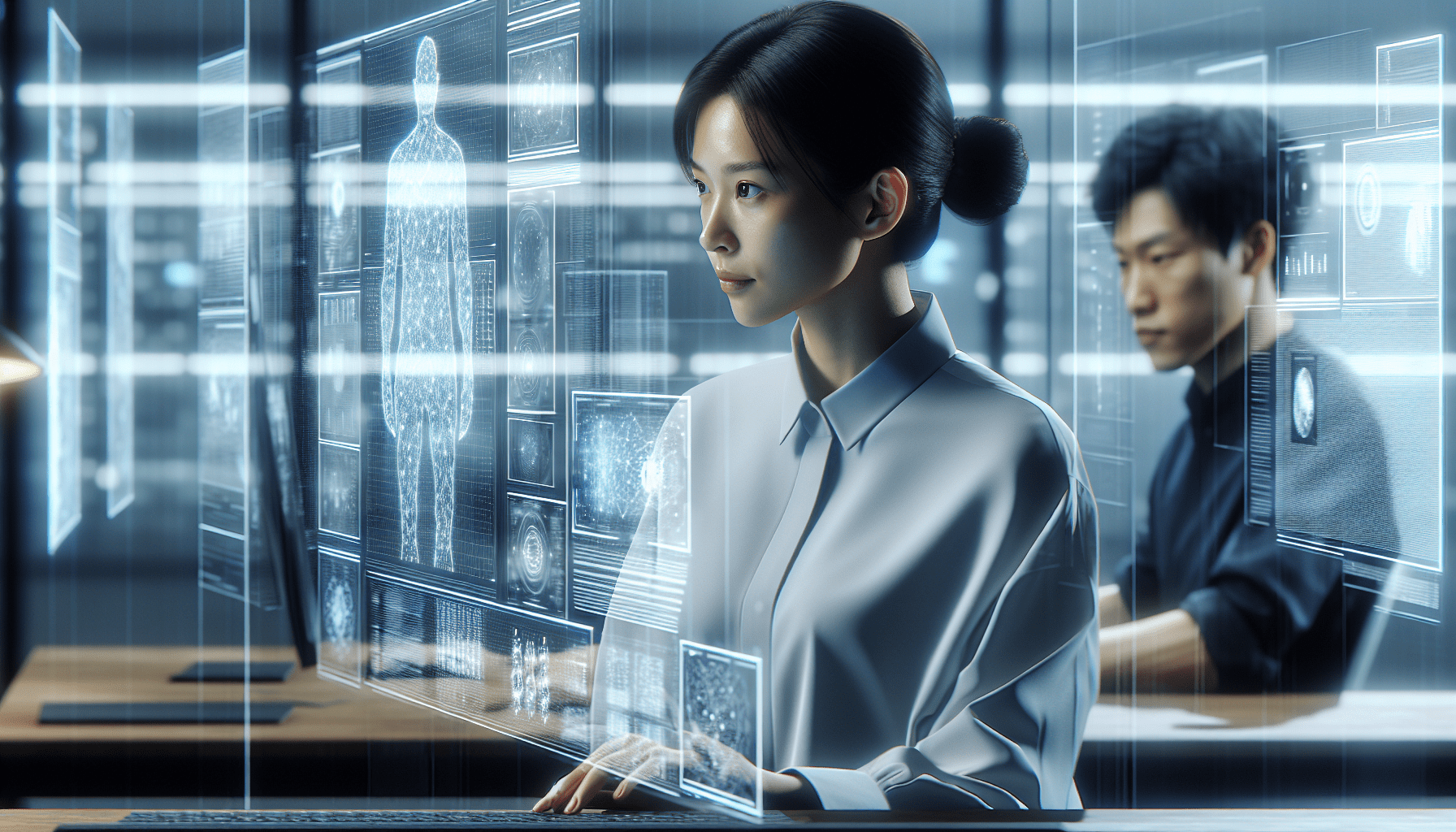
Increased Adoption of Human-in-the-Loop Systems
As the demand for ethical AI practices continues to grow, we can expect to see an increased adoption of human-in-the-loop systems in the software development industry. These systems provide a valuable framework for integrating human judgment into AI processes, ensuring that decisions are fair, accurate, and free from bias. By incorporating human oversight, companies can improve the reliability and trustworthiness of their AI applications.
For marketing managers, digital strategists, and executives, understanding how to implement human-in-the-loop systems will become increasingly important. These systems offer a practical solution for addressing biases in AI-driven advertising strategies, ultimately enhancing ROI and customer satisfaction. As more companies recognize the benefits of human-in-the-loop systems, we can expect to see widespread adoption across various industries.
Overall, the increased adoption of human-in-the-loop systems represents a significant trend in bias mitigation. By integrating human oversight into AI processes, companies can ensure that their AI systems are fair, ethical, and effective. This leads to better business outcomes and increased trust with customers and stakeholders.
Advancements in Bias Detection and Mitigation Technologies
As the field of AI continues to evolve, we can expect to see significant advancements in bias detection and mitigation technologies. These technologies will play a crucial role in identifying and addressing biases in AI systems, ensuring that decisions are fair and equitable. By leveraging advanced technologies, companies can improve the accuracy and fairness of their AI applications.
For companies in the software development industry, staying informed about the latest advancements in bias detection and mitigation technologies will be essential. These technologies offer valuable tools for improving the effectiveness of AI-driven advertising strategies, ultimately enhancing ROI and customer satisfaction. As new technologies emerge, companies will have more options for addressing biases and ensuring the ethical deployment of AI systems.
Overall, advancements in bias detection and mitigation technologies represent a significant trend in the field of AI. By leveraging these technologies, companies can improve the fairness and accuracy of their AI applications, leading to better business outcomes and increased trust with customers and stakeholders.
Growing Emphasis on Ethical AI Practices
As AI systems become more prevalent, there is a growing emphasis on ethical AI practices. Companies are increasingly recognizing the importance of ensuring that their AI applications are fair, equitable, and free from bias. This emphasis on ethical AI practices is driven by the need to build trust with customers and stakeholders, as well as the growing demand for ethical AI deployment.
Understanding the importance of ethical AI practices will become increasingly important for marketing managers, digital strategists, and executives. By implementing ethical guidelines, policies, and procedures, companies can ensure that their AI systems are fair and representative. This approach not only improves the quality of AI systems but also enhances the overall effectiveness of AI applications.
Overall, the growing emphasis on ethical AI practices represents a significant trend in the field of AI. By prioritizing ethical considerations, companies can build trust with their customers and stakeholders, ultimately leading to better business outcomes and increased trust with customers and stakeholders.
Increased Collaboration Between Industry and Academia
As the field of AI continues to evolve, we can expect to see increased collaboration between industry and academia. This collaboration will play a crucial role in advancing the field of AI and addressing the challenges of bias mitigation. By working together, industry and academia can develop new strategies and technologies to ensure the fairness and accuracy of AI systems.
For companies in the software development industry, collaborating with academic institutions can provide valuable insights and expertise for addressing biases in AI systems. By leveraging academia’s knowledge and resources, companies can improve the effectiveness of their AI-driven advertising strategies, ultimately enhancing ROI and customer satisfaction.
Overall, increased collaboration between industry and academia represents a significant trend in the field of AI. By working together, industry and academia can develop new strategies and technologies for ensuring the fairness and accuracy of AI systems, leading to better business outcomes and increased trust with customers and stakeholders.
Emergence of New Regulatory Frameworks for AI
As AI systems become more prevalent, we can expect to see the emergence of new regulatory frameworks for AI. These frameworks will play a crucial role in ensuring the ethical deployment of AI systems and addressing the challenges of bias mitigation. By establishing clear guidelines and standards, regulatory frameworks can help companies ensure that their AI applications are fair and equitable.
Staying informed about new regulatory frameworks will be essential for software development companies. These frameworks offer valuable guidance for addressing biases in AI systems and ensuring the ethical deployment of AI applications. By complying with regulatory frameworks, companies can build trust with their customers and stakeholders, ultimately leading to better business outcomes.
Overall, the emergence of new regulatory frameworks for AI represents a significant trend in the field of AI. By establishing clear guidelines and standards, regulatory frameworks can help companies ensure that their AI applications are fair and equitable, leading to better business outcomes and increased trust with customers and stakeholders.
Conclusion
In conclusion, the integration of human-in-the-loop systems for bias mitigation is a crucial strategy for ensuring the fairness and accuracy of AI-driven systems. By incorporating human oversight, companies can improve the reliability and trustworthiness of their AI applications, ultimately enhancing ROI and customer satisfaction. As the demand for ethical AI practices continues to grow, understanding how to implement human-in-the-loop systems will become increasingly important for marketing managers, digital strategists, and executives in the software development industry.
By addressing the challenges of data bias, algorithmic bias, lack of transparency, and ethical considerations, companies can ensure that their AI systems are fair, ethical, and effective. By implementing the step-by-step solutions outlined in this article, companies can improve the fairness and accuracy of their AI applications, leading to better business outcomes and increased trust with customers and stakeholders.
More Information
- Algorithmic Bias Detection and Mitigation: Best Practices and Policies to Reduce Consumer Harms – A comprehensive guide on best practices for reducing bias in AI systems.
- Algorithmic Bias Detection and Mitigation: Best Practices and Policies to Reduce Consumer Harms | Brookings – An article discussing the importance of bias detection and mitigation in AI systems.
- Mitigating AI/ML Bias in Context | NCCoE – A project focused on developing guidance for bias mitigation in AI systems.
Disclaimer
This is an AI-generated article for educational purposes and does not intend to give advice. The goal is to inspire further research and exploration of the topics covered in the article.
- The Role of Color in Brand Identity - 10/23/24
- Human-in-the-Loop for Bias Mitigation - 10/16/24
- Challenges in Implementing Federated Learning in Ad Tech - 10/09/24