Table of Contents
Understanding ML Threat Prediction in Advertising
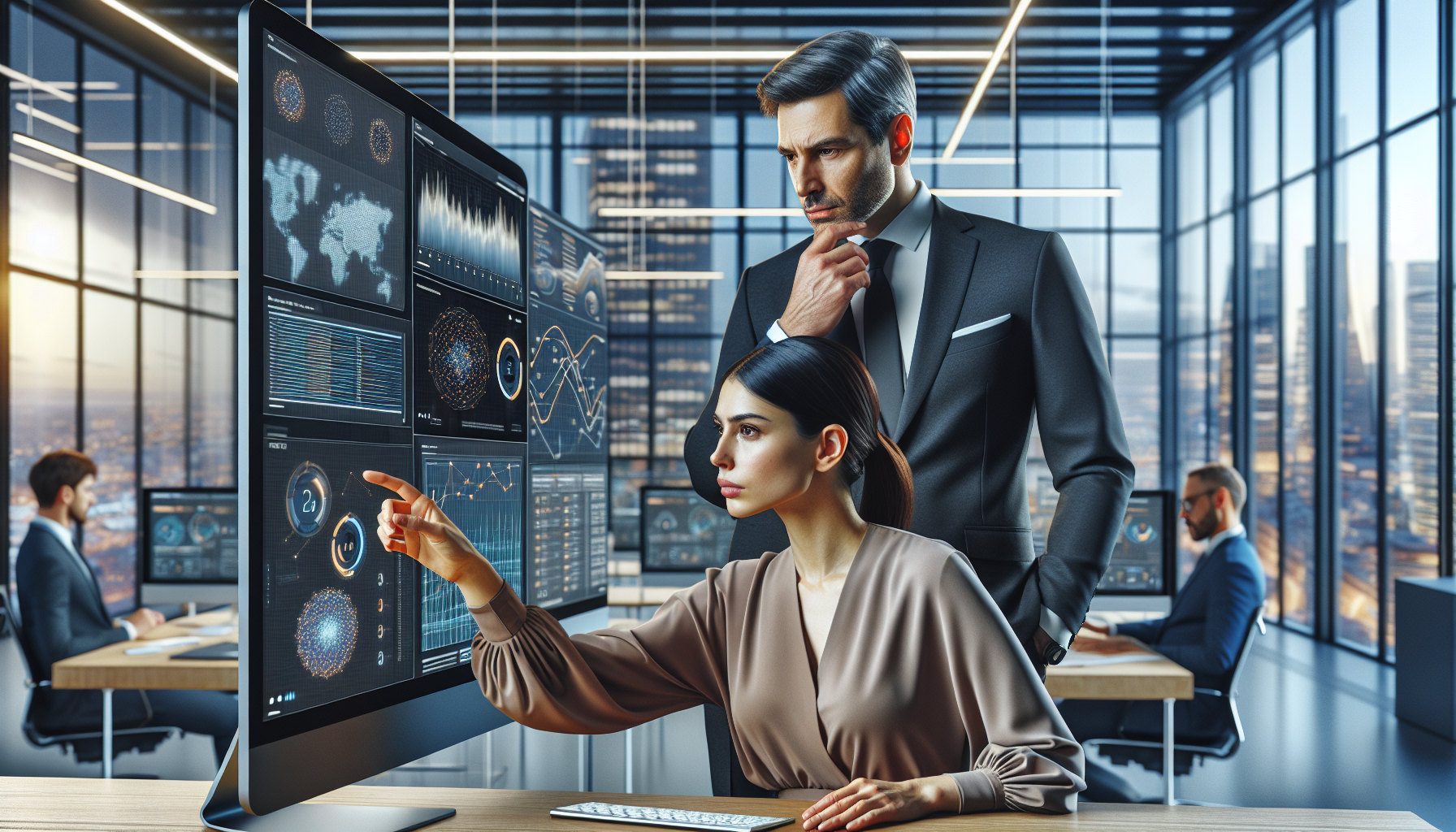
Overview of ML Threat Prediction
ML threat prediction in advertising is a burgeoning field that leverages machine learning techniques to identify and mitigate potential threats in advertising campaigns. This includes fraud detection, privacy breaches, and malicious ad content.
By analyzing vast amounts of data and recognizing patterns that may indicate suspicious activity, ML models can preemptively alert businesses to potential threats, safeguarding both the brand and its audience.
Data sources for ML threat prediction in advertising are diverse, ranging from user interaction data and ad traffic data to real-time bidding information. Integrating these data sources provides a holistic view of the advertising landscape, enabling more accurate threat detection.
Challenges in this field include the dynamic nature of advertising threats, the need for real-time processing, and maintaining user privacy while collecting and analyzing data. Advanced ML models are continuously being developed to address these challenges, making threat prediction more robust and reliable.
Implementing ML threat prediction can enhance security, improve user trust, and improve compliance with regulatory standards, all of which contribute to a more secure and efficient digital advertising ecosystem.
Technological Foundations
ML models used in threat prediction typically involve supervised learning, where models are trained on labeled datasets that define ‘normal’ and ‘threatening’ advertising activities. Techniques such as neural networks, decision trees, and clustering are commonly employed to classify and predict threats.
Feature engineering is a critical step in enhancing the performance of ML models. In the context of ad threat prediction, features might include the number of clicks per second, IP address patterns, and unusual changes in account behavior.
Model training involves adjusting the algorithms to accurately predict threats based on historical data. This phase is crucial for determining the effectiveness of the model in real-world scenarios.
Continuous learning is essential as new types of threats emerge. ML models must be regularly updated and retrained with new data to adapt to the evolving digital landscape and maintain high accuracy in threat detection.
Challenges in Protecting Digital Advertising
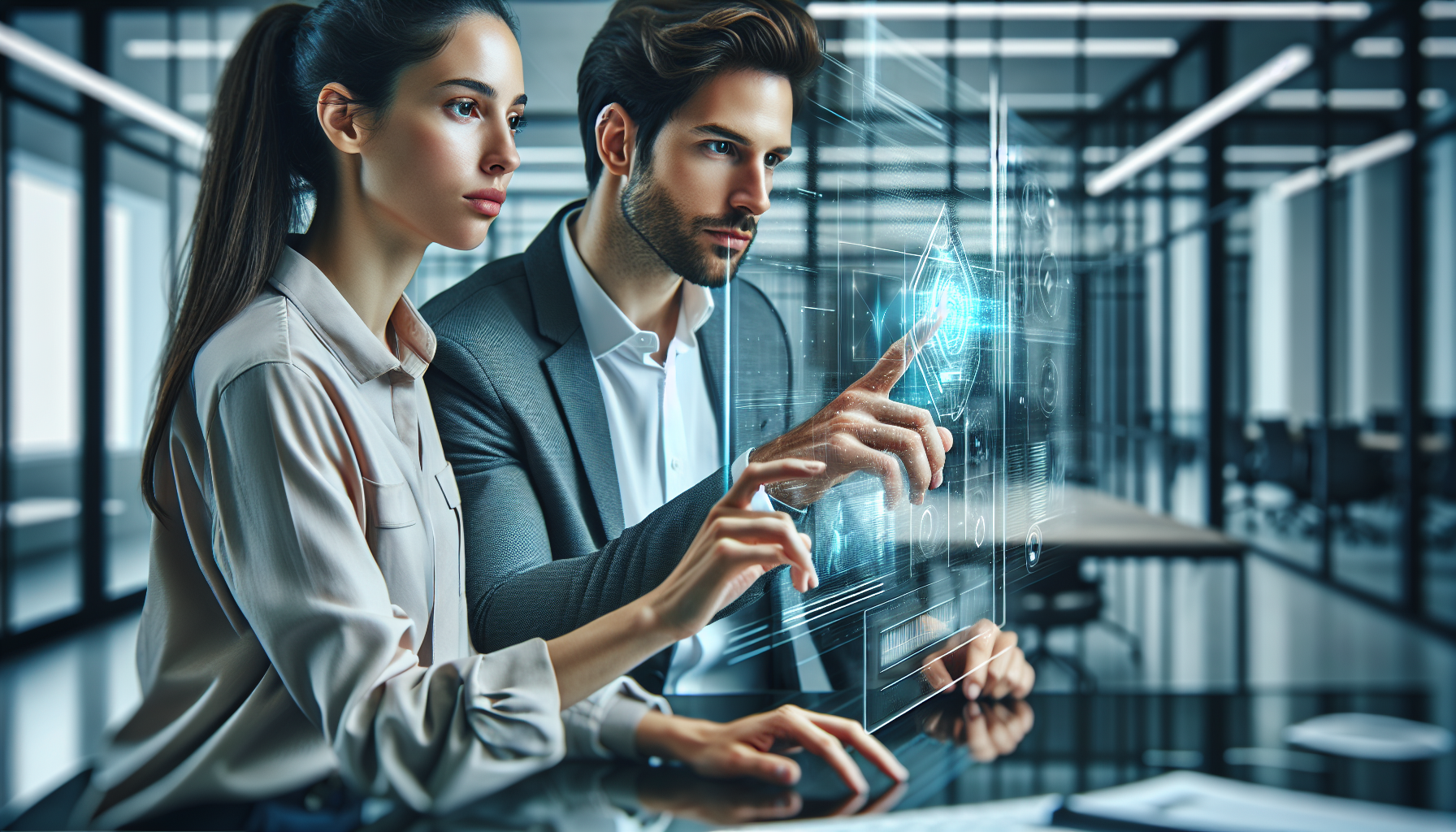
Identifying Hidden Threats in Real-Time Bidding
In the fast-paced environment of digital advertising, particularly within real-time bidding (RTB) systems, identifying and mitigating threats instantaneously poses a significant challenge.
RTB involves automated auctions, where advertising inventory is bought and sold in real-time on a per-impression basis. While efficient, this system opens up numerous vulnerabilities where malicious actors can exploit speed and automation to introduce threats such as ad fraud and malware distribution.
The complexity of the RTB ecosystem, involving multiple parties like advertisers, publishers, and ad exchanges, further complicates threat detection. Each participant in the RTB chain can potentially be a vector for security breaches, making comprehensive monitoring and threat prediction crucial.
Moreover, the sheer volume of transactions and the speed at which they occur make it impractical for traditional security measures to cope effectively. This is where ML models come into play, offering the ability to analyze vast datasets quickly, recognize patterns indicative of threats, and implement protective measures in real time.
Another critical challenge is ensuring user privacy while conducting deep analysis for threat detection. Advertisers and platforms must balance aggressive threat detection techniques with stringent privacy standards to avoid alienating users and violating regulations like GDPR.
Implementing Advanced ML Solutions
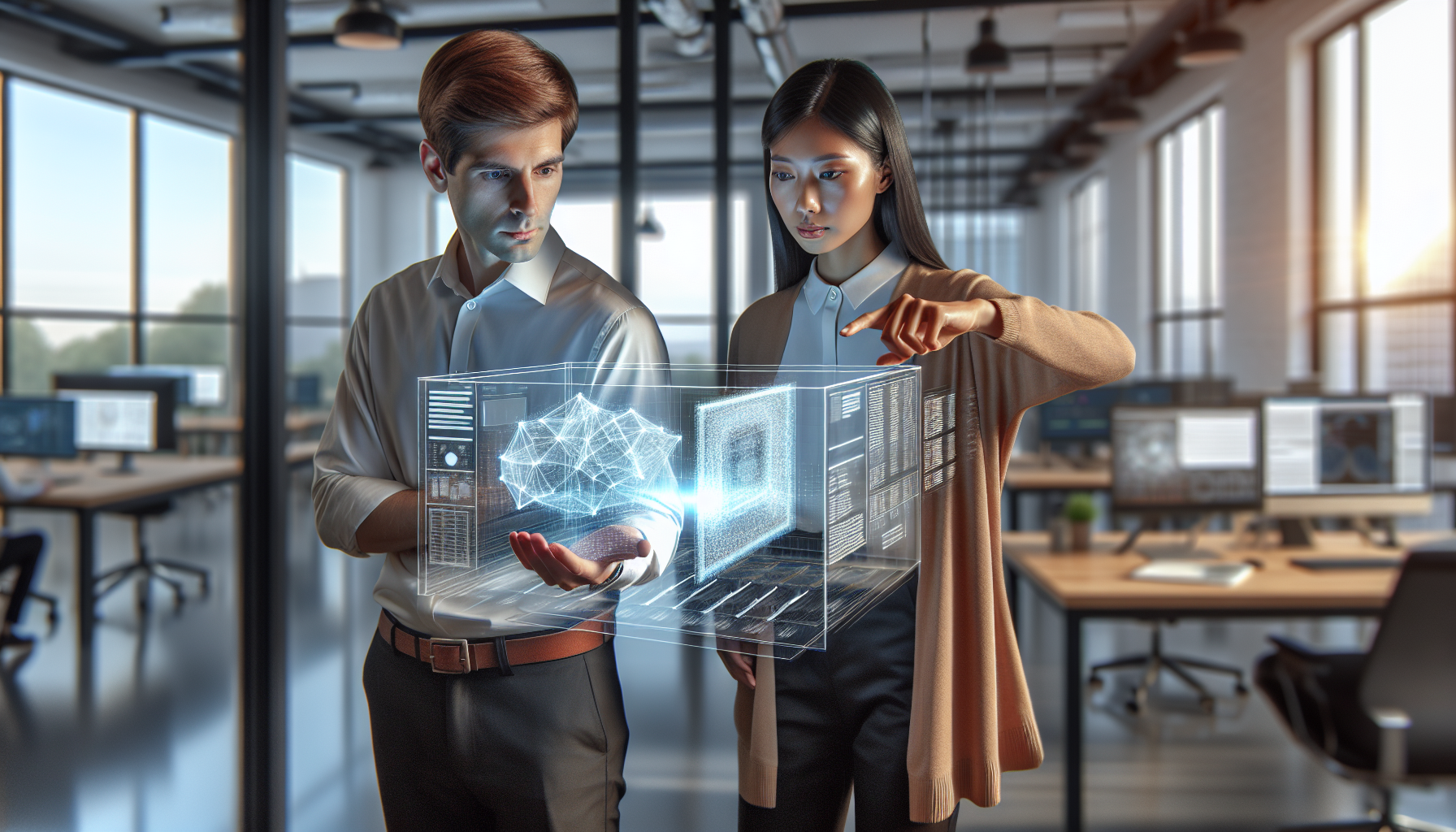
Step-by-Step Approach to Enhancing RTB Security
To address the challenges posed by real-time bidding in digital advertising, implementing an advanced ML-based threat prediction system involves several key steps:
- Data Collection: Gather and preprocess data from various sources within the RTB ecosystem, ensuring privacy compliance.
- Feature Selection: Identify and select features that are most indicative of potential threats based on historical data and expert input.
- Model Development: Develop and train ML models using selected features. Techniques like neural networks or ensemble methods may be employed depending on the complexity of the threat patterns.
- Real-Time Implementation: Integrate the trained ML model into the RTB system to analyze bidding data in real time, allowing for immediate detection and mitigation of threats.
- Continuous Monitoring and Updating: Regularly monitor the ML system’s performance, updating and retraining the model with new data and adjusting to new threat patterns and tactics used by malicious actors.
Frequently Asked Questions
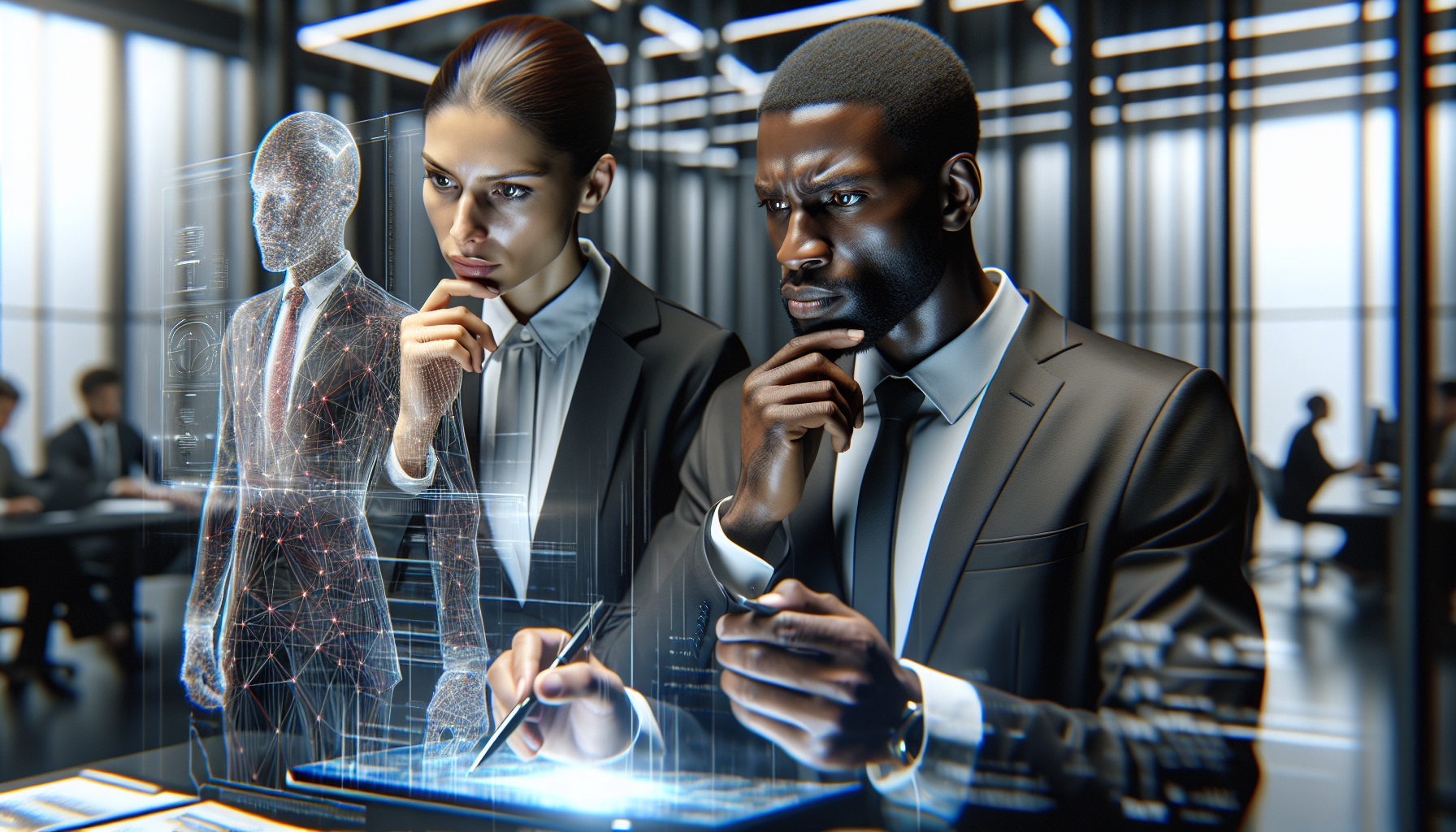
What is ML threat prediction in advertising?
ML threat prediction in advertising refers to the use of machine learning techniques to identify and mitigate potential threats in digital advertising campaigns, such as fraud, privacy breaches, and malicious content.
How do ML models detect threats in real-time bidding?
ML models detect threats in real-time bidding by analyzing bidding data in real-time, identifying patterns indicative of malicious activity, and triggering alerts or preventive measures.
What are the benefits of using ML for threat prediction in advertising?
Benefits include enhanced security, increased user trust, and compliance with regulatory standards, leading to a safer and more efficient digital advertising ecosystem.
Can ML models ensure user privacy while detecting threats?
Yes, ML models can be designed to respect user privacy by implementing data anonymization and ensuring compliance with privacy regulations like GDPR during the data analysis process.
What are the challenges of implementing ML threat prediction?
Challenges include the dynamic nature of advertising threats, the need for real-time processing, and balancing aggressive threat detection with user privacy concerns.
Future of ML in Advertising Security
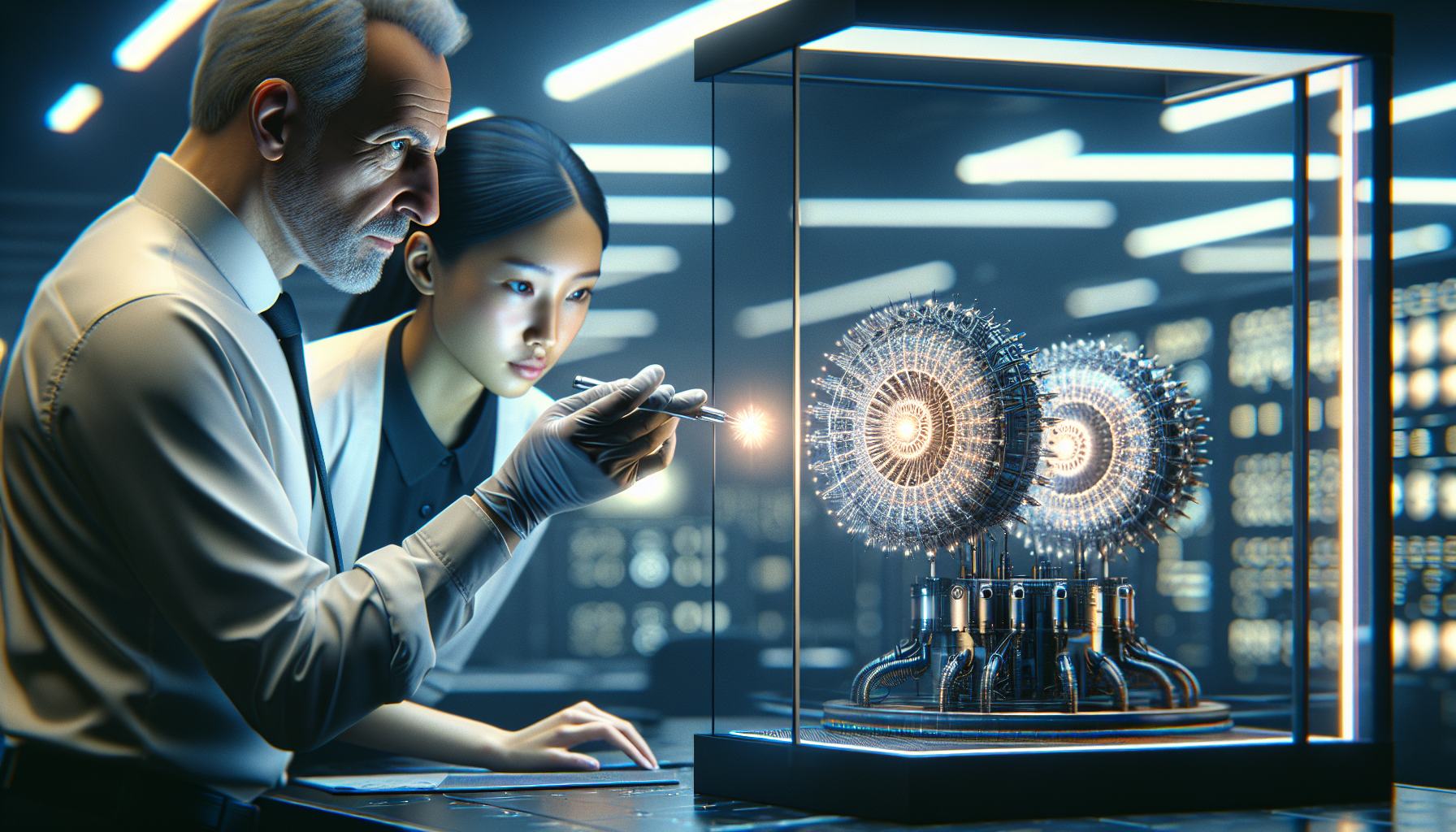
As digital advertising continues to evolve, so too will the techniques used to secure it. Here are five predictions for the future of ML in advertising security:
- Increased Automation: ML models will become more autonomous in detecting and responding to threats, reducing the need for human intervention.
- Greater Integration: ML-based security solutions will be more deeply integrated into advertising platforms, providing seamless protection throughout the advertising process.
- Enhanced Personalization: Security measures will become more personalized, using ML to tailor protection strategies to individual user patterns and behaviors.
- Advanced Anomaly Detection: ML models will develop enhanced capabilities to detect anomalies and unusual patterns more effectively, even in highly complex datasets.
- Focus on Privacy: There will be a stronger emphasis on developing ML models that provide robust security without compromising user privacy, aligning with global data protection regulations.
Disclaimer
This article is AI-generated for educational purposes and does not intend to give advice or recommend its implementation. It aims to inspire readers to research and delve deeper into the topics covered.