Table of Contents
Understanding AI in RTB Fraud Detection
Key Points
AI fraud detection in RTB is crucial for maintaining the integrity and efficiency of digital advertising ecosystems. Here are the key takeaways:
- AI enhances accuracy and speed in identifying fraudulent activities by analyzing patterns and anomalies in large datasets.
- Techniques such as machine learning, deep learning, and natural language processing are employed to scrutinize various signals like user behavior and geolocation.
- AI models are trained using supervised, unsupervised, or reinforcement learning to adapt and improve over time.
- Despite its advantages, AI in RTB fraud detection faces challenges such as data quality, model complexity, and ethical concerns.
- Best practices include ensuring data integrity, adhering to privacy standards, and maintaining transparency in AI decision-making processes.
Technological Foundations
At the core of AI fraud detection in RTB are advanced algorithms that process and analyze vast amounts of data in real time. These algorithms are capable of learning from data inputs and continuously improving, making them highly effective at detecting fraudulent patterns that would elude human analysts.
The technology leverages various AI disciplines, including machine learning (ML), deep learning (DL), and natural language processing (NLP). Each of these plays a pivotal role in interpreting the data collected during the bidding process, from bid requests to user interaction data.
For instance, ML models can be trained to recognize the digital footprint of known fraud techniques such as location spoofing or irregular clicking patterns. Similarly, DL can analyze the visual content of ads to detect sophisticated frauds like ad stacking or pixel stuffing.
NLP techniques help in assessing the context of content and user interactions, further refining the fraud detection process. By integrating these AI technologies, RTB platforms can significantly enhance their fraud detection capabilities.
Implementation in the Industry
The implementation of AI for fraud detection in RTB is seen across various platforms and companies in the ad tech industry. Major RTB platforms integrate AI systems to monitor and analyze every bid in real time, ensuring that each transaction is legitimate.
Companies like Adjust and Sonobi are at the forefront, utilizing AI to safeguard their bidding environments. These platforms use AI not only to detect fraud but also to optimize bidding strategies and maximize ROI for advertisers.
By implementing AI, these companies can offer more secure and efficient advertising options, attracting more business while protecting existing investments. The continuous evolution of AI technology also promises further enhancements in fraud detection accuracy and speed.
As AI technology advances, its integration into RTB systems is becoming more sophisticated, allowing for real-time responses to emerging fraud threats and adapting to new fraudulent tactics as they are developed.
The Challenge of Scaling AI in RTB Systems
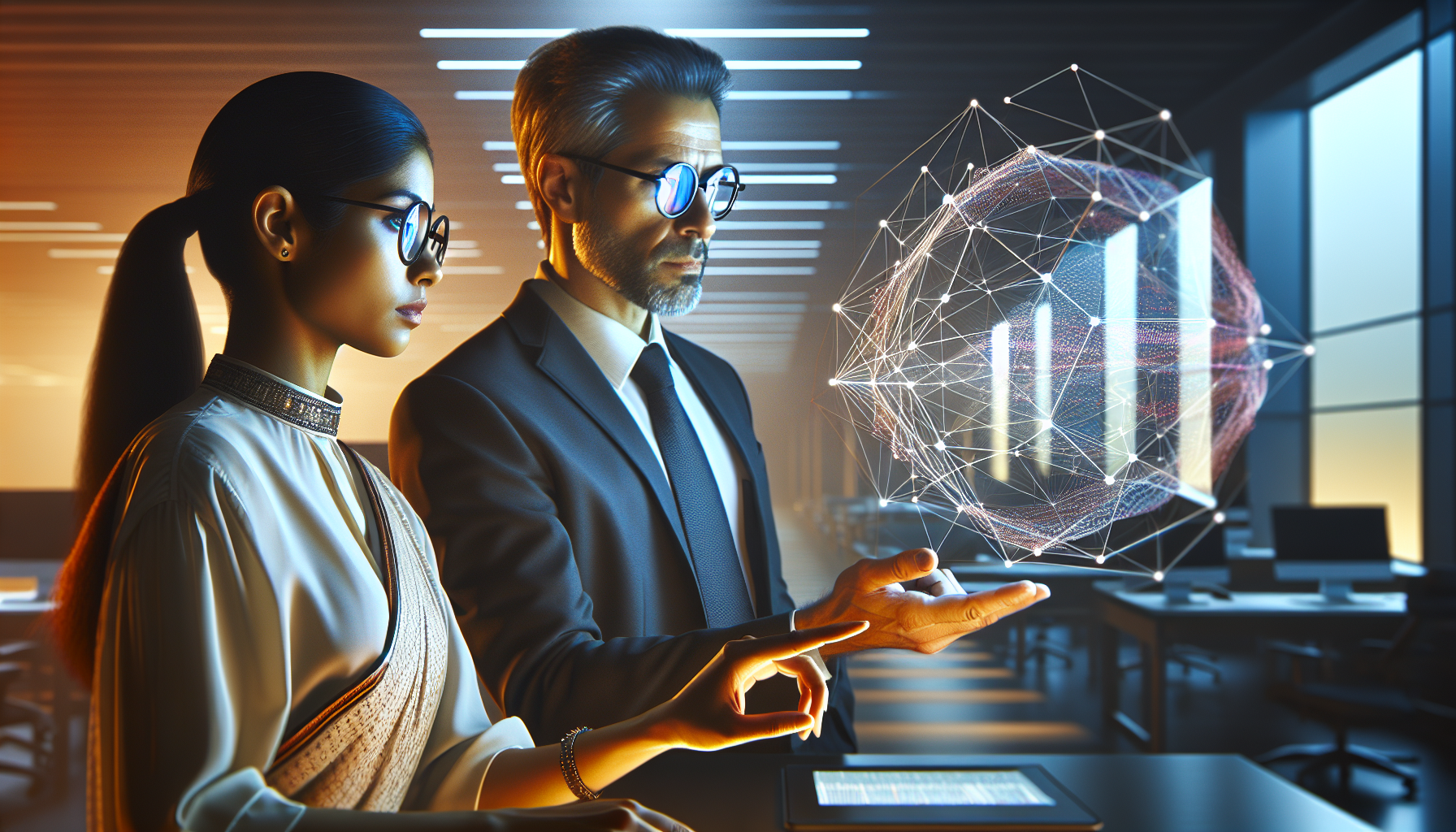
Complexity and Resource Intensity
One of the most significant challenges in implementing AI for fraud detection in RTB is the complexity and resource intensity of AI systems. These systems require substantial computational power to process and analyze the vast amounts of data generated in real-time bidding environments.
Moreover, the development and maintenance of AI models necessitate a team of skilled data scientists and engineers. These professionals must continuously update and refine the models to keep up with the evolving tactics of fraudsters, which can be a costly and resource-intensive endeavor.
Additionally, the integration of AI into existing RTB infrastructures often requires significant architectural changes. These changes can disrupt operations and lead to downtime, which is detrimental in an industry where real-time processing is critical.
Furthermore, the complexity of AI models can make them opaque, leading to challenges in understanding and explaining the decisions made by these systems. This lack of transparency can raise concerns among stakeholders regarding accountability and fairness in ad bidding and placement.
Optimizing AI Deployment in RTB
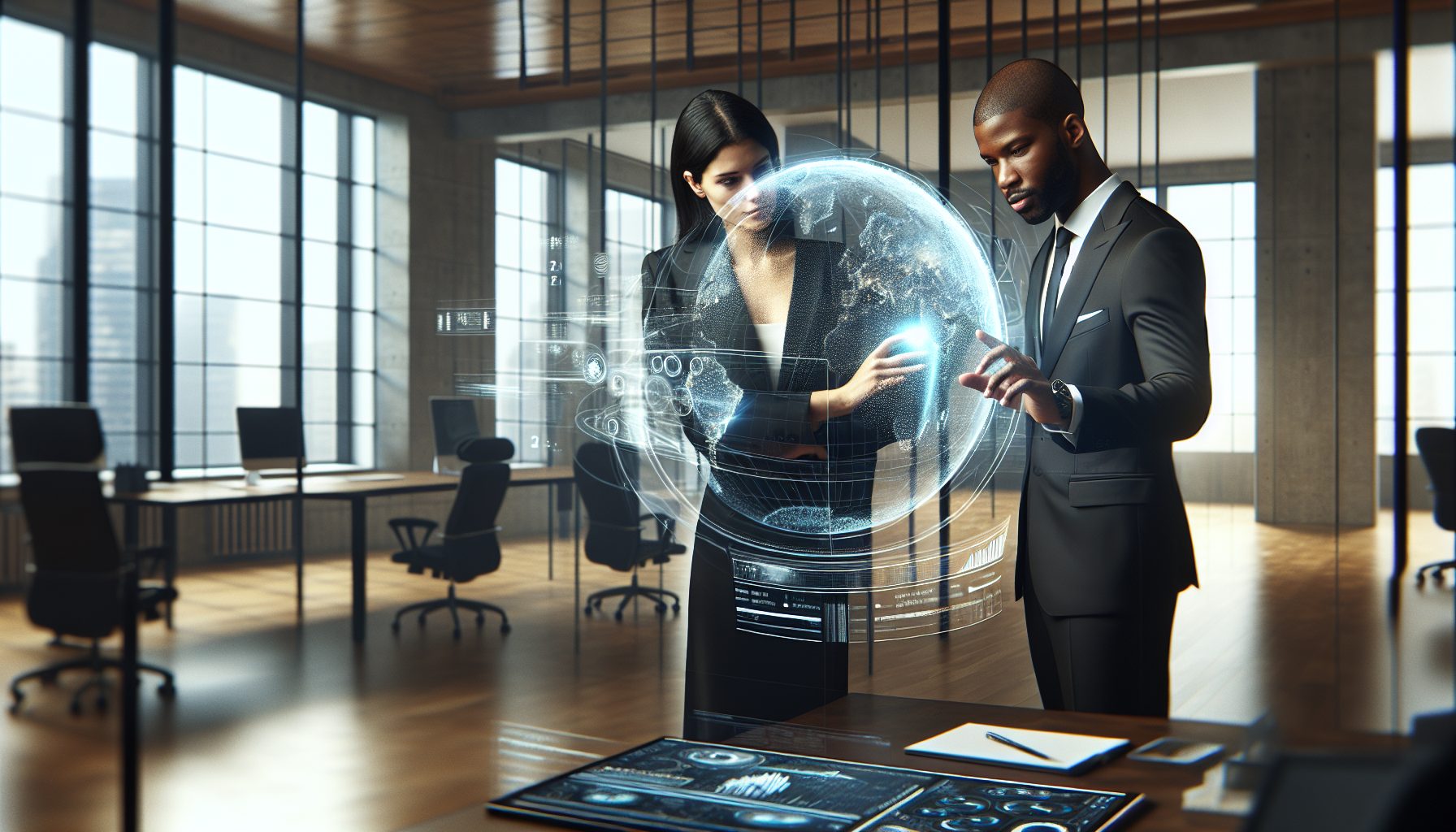
Strategic Implementation
To effectively deploy AI in RTB systems for fraud detection, it is essential to adopt a strategic approach that balances complexity with performance. This involves selecting the right AI models that are both efficient and scalable while being capable of handling the specific types of fraud encountered in RTB.
The deployment should start with a pilot phase, where AI is implemented in a controlled segment of the RTB system. This allows for monitoring the AI’s performance and making necessary adjustments before a full-scale rollout.
It is also crucial to ensure that the AI systems are integrated seamlessly with the existing RTB infrastructure to minimize disruptions. This requires close collaboration between AI specialists and RTB engineers to align the technologies effectively.
Regular training and updates of AI models are necessary to keep them effective against new and evolving fraud tactics. This involves not only retraining the models with new data but also refining the algorithms to improve accuracy and reduce false positives.
Frequently Asked Questions
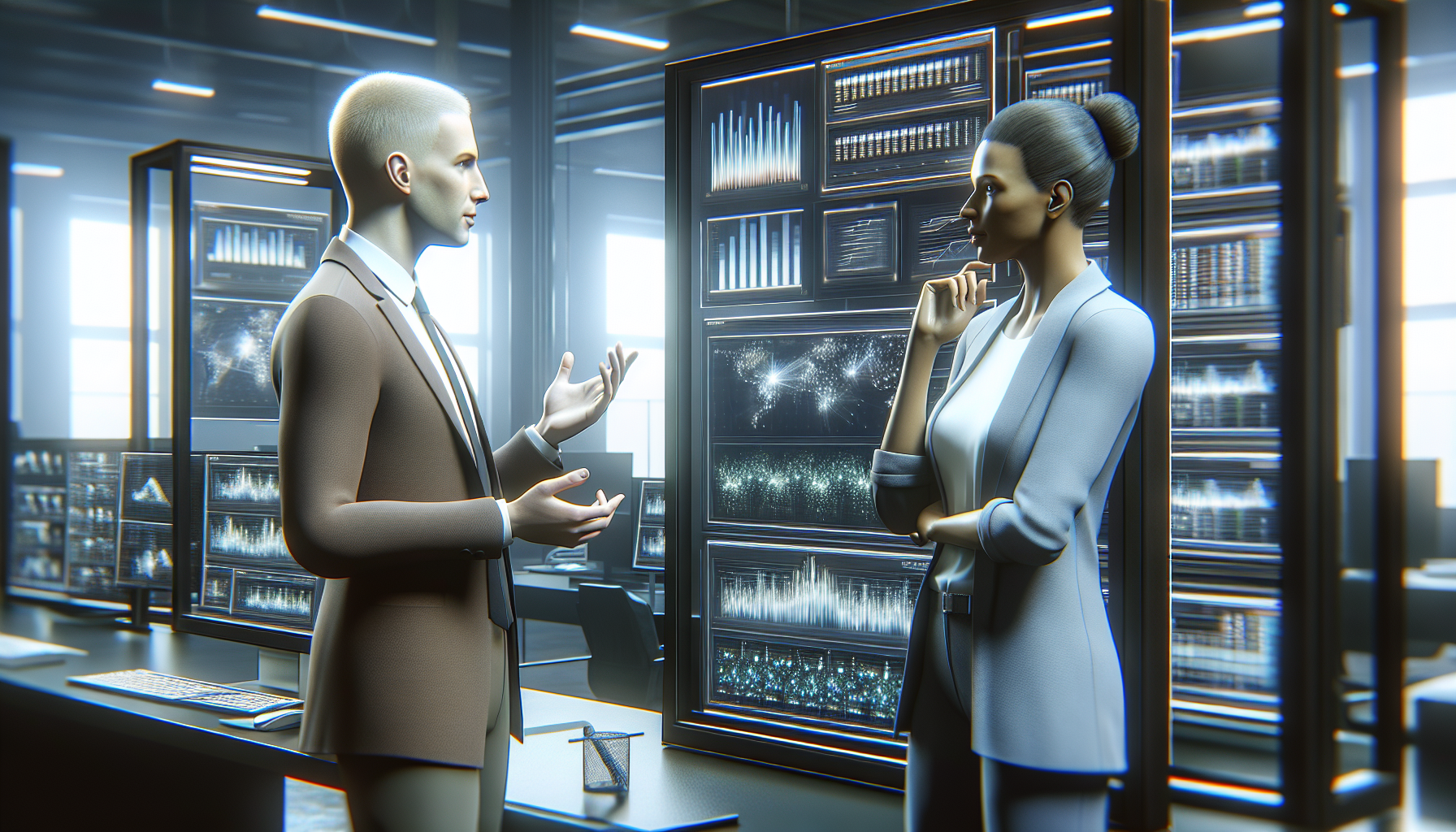
What is real-time bidding?
Real-time bidding is an automated digital auction process that allows advertisers to bid on ad impressions in real time, as a user visits a website. This process enables more precise and efficient ad placements.
How does AI enhance RTB fraud detection?
AI improves fraud detection in RTB by analyzing vast amounts of data to identify patterns and anomalies that indicate fraudulent activity, thus enhancing the accuracy and speed of detection.
What are the main challenges of using AI in RTB?
The main challenges include the complexity of AI models, the high resource requirements for training and running these models, and the need for continuous updates to keep up with new fraud tactics.
Can AI completely eliminate fraud in RTB?
While AI significantly reduces the incidence of fraud in RTB, it cannot completely eliminate it. Continuous advancements in AI are necessary to keep up with the sophisticated tactics of fraudsters.
What are the best practices for implementing AI in RTB?
Best practices include choosing the right AI models, integrating AI smoothly with existing systems, continuously training and updating the models, and maintaining transparency in AI-driven decisions.
Looking Ahead: The Future of AI in RTB Fraud Detection
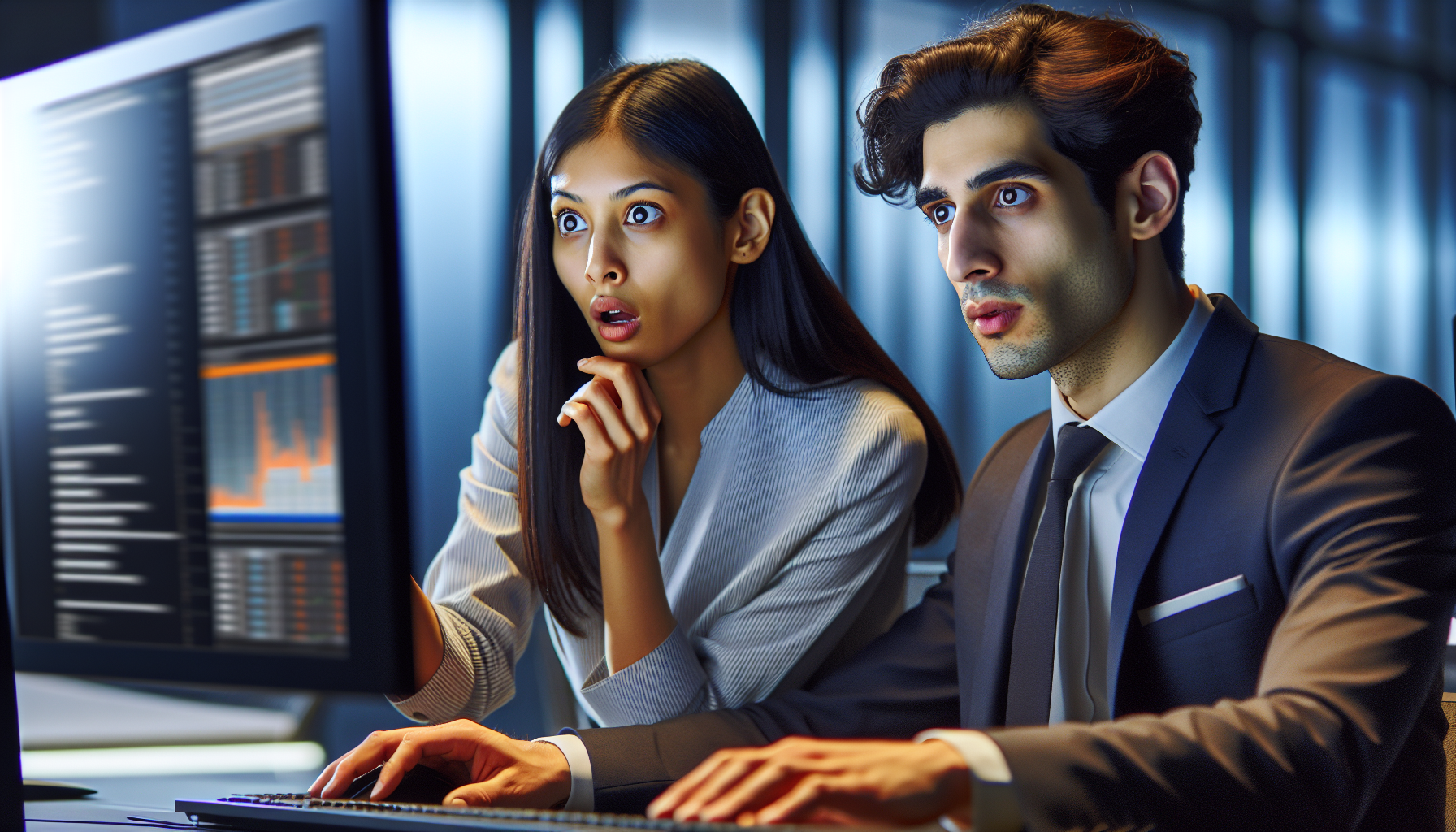
As we look to the future, the role of AI in RTB fraud detection is set to expand significantly. Here are five predictions:
- Increased Adoption of AI: More companies will adopt AI technologies to combat fraud, driven by the increasing sophistication of fraud tactics.
- Advancements in AI Technology: AI models will become more advanced, with better capabilities to detect and prevent fraud in real time.
- Greater Integration with Other Technologies: AI will be increasingly integrated with other technologies such as blockchain to enhance security and transparency in RTB.
- Enhanced Regulatory Compliance: AI will play a crucial role in ensuring compliance with evolving privacy and security regulations in digital advertising.
- Broader Ethical Considerations: There will be a greater focus on the ethical implications of using AI in advertising, including issues of bias and fairness.
References
- What is real-time bidding (RTB)? | Adjust
- The Role of Artificial Intelligence in Programmatic Advertising | Sonobi
Disclaimer
This article is AI-generated for educational purposes and does not intend to provide advice or recommend its implementation. The goal is to inspire readers to research and delve deeper into the topics covered.