Table of Contents
Understanding Predictive Analytics
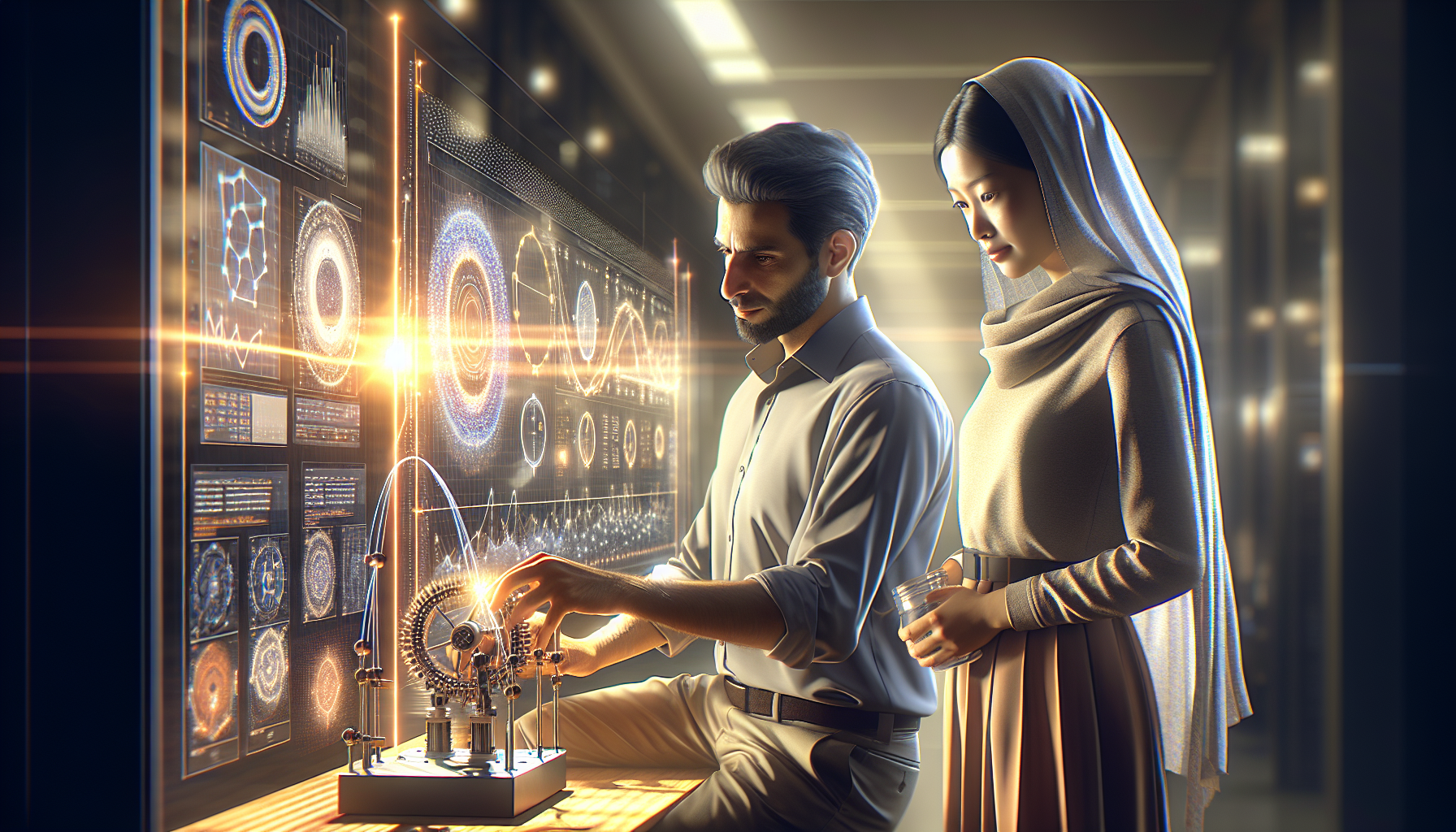
Key Points
- Predictive analytics utilizes data mining, predictive modeling, and machine learning to forecast future behaviors and trends.
- It enhances marketing strategies by providing insights into customer preferences and future actions.
- Businesses can use predictive analytics to optimize resource allocation, improving ROI on marketing campaigns.
- It allows for more personalized customer experiences by anticipating needs and preferences.
- Real-time data processing with predictive analytics helps businesses make swift, informed decisions.
Core Concepts
Predictive analytics is a branch of advanced analytics that uses historical data, statistical algorithms, and machine learning techniques to identify the likelihood of future outcomes based on historical data. It is widely used in marketing to enhance decision-making processes, from customer segmentation to optimizing marketing campaigns and improving customer service.
The process involves collecting data, developing a statistical model, and making predictions. The model is then validated and revised as more data becomes available. In marketing, this approach helps in understanding and predicting customer behavior, which can lead to more effective marketing strategies.
Technological Framework
The technological infrastructure for implementing predictive analytics involves a combination of data management solutions, statistical analysis tools, and machine learning algorithms. Data is gathered from various sources, cleaned, and processed to form a dataset that can be analyzed to build predictive models.
Tools like R, Python, SAS, and specialized software like IBM SPSS Modeler are used to create and train models to predict customer behavior or outcomes. These tools provide the capability to handle large datasets and perform complex computations necessary for predictive analysis.
Challenges in Ad Tech AI Advertising
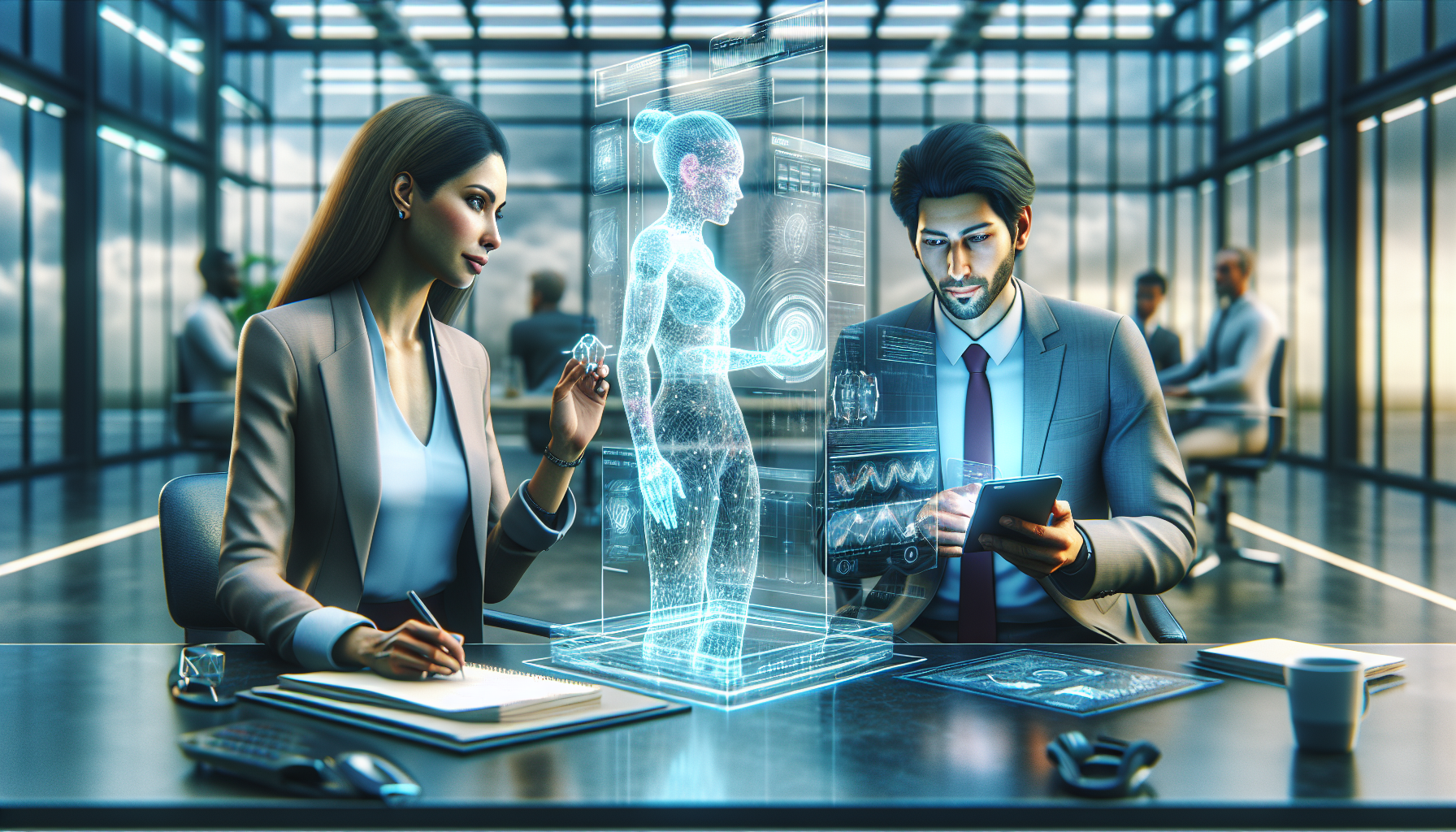
Complexity in User Data Integration
Integrating user data from diverse platforms presents significant challenges in ad tech. Marketers often struggle with disparate data formats, incomplete data sets, and data collection latency, which can lead to inaccurate targeting and suboptimal user engagement.
For instance, a marketer might have access to extensive data from social media interactions but lacks integration with e-commerce behavior or offline purchase data. This incomplete picture can skew predictive models, leading to less effective advertising campaigns.
Privacy Concerns and Data Security
With the increasing use of predictive analytics in marketing, concerns about privacy and data security are more prominent. Users are becoming more conscious of their data privacy and are often reluctant to share personal information, which is crucial for building predictive models.
Moreover, data breaches and other security risks can compromise user trust and affect the reliability of predictive analytics. Ensuring data security and addressing privacy concerns are therefore critical for marketers using predictive analytics in advertising.
Adapting to Rapidly Changing Technologies
The fast pace of technological advancements in ad tech poses another challenge. Marketers must continuously learn and adapt to new tools and technologies to stay competitive. This requires ongoing training and resources, which can be a significant investment.
Staying updated with the latest predictive analytics tools and techniques is essential for marketers to leverage the full potential of AI in advertising. However, this can be daunting due to the technical complexity and the fast-evolving nature of the technology.
Strategies to Enhance User Engagement with Predictive Analytics
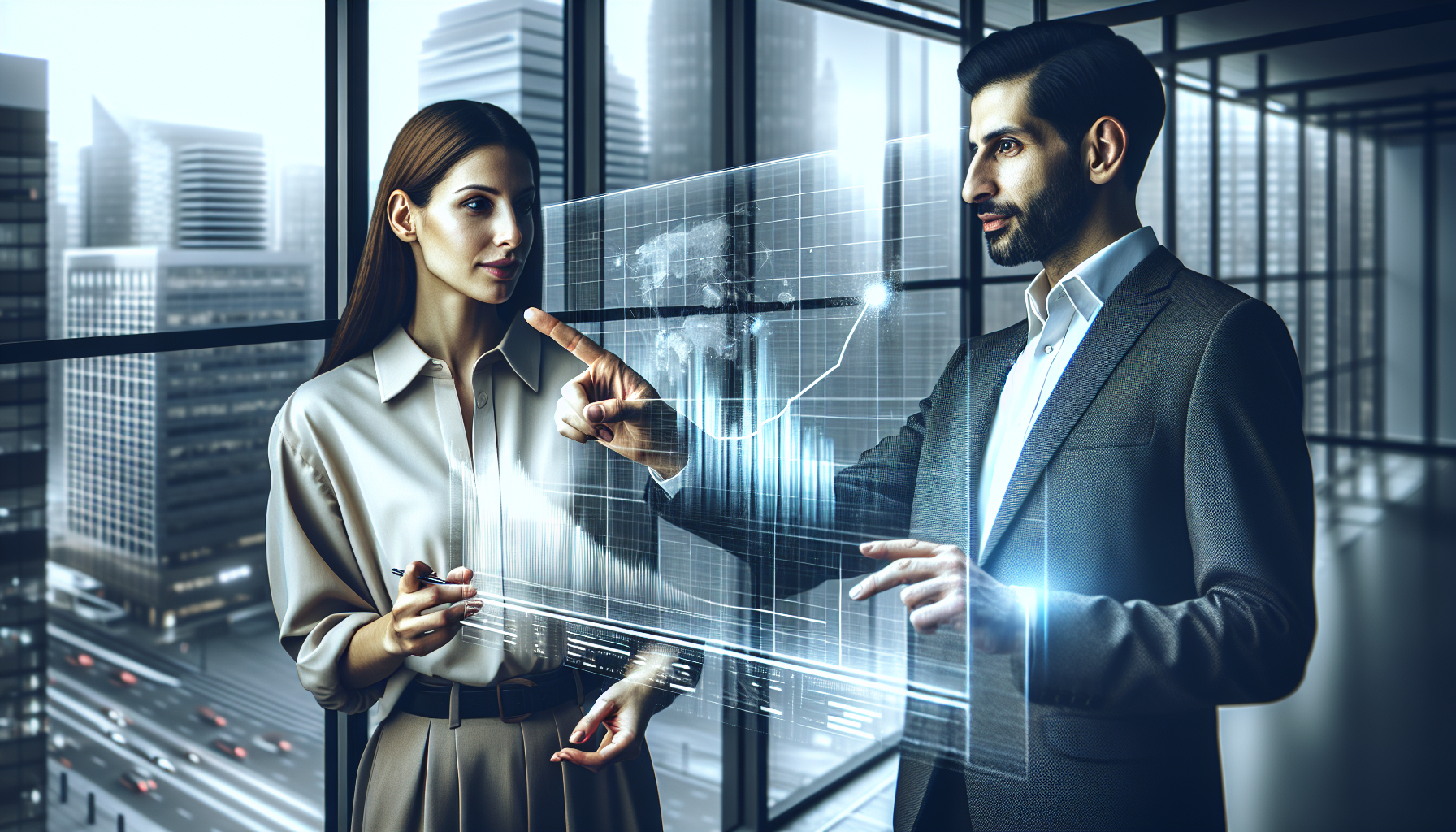
Step 1: Data Collection and Integration
Begin by integrating data from all available sources. This includes web behavior, social media interactions, transactional data, and any other relevant user information. Ensuring the data is clean and organized is crucial for accurate predictions.
Step 2: Model Development
Develop a predictive model using statistical tools and machine learning algorithms. The model should be tailored to predict user behaviors that are most relevant to your marketing goals, such as purchase likelihood, product preferences, or engagement levels.
Step 3: Apply Insights to Marketing Strategies
Use the insights gained from the predictive model to tailor your marketing strategies. This could involve personalizing ad content, optimizing send times for marketing communications, or more effectively targeting specific user segments.
Step 4: Continuous Refinement
Continuously refine your predictive model based on new data and feedback from marketing outcomes. This iterative process helps improve the accuracy of your predictions and the effectiveness of your marketing strategies.
Frequently Asked Questions
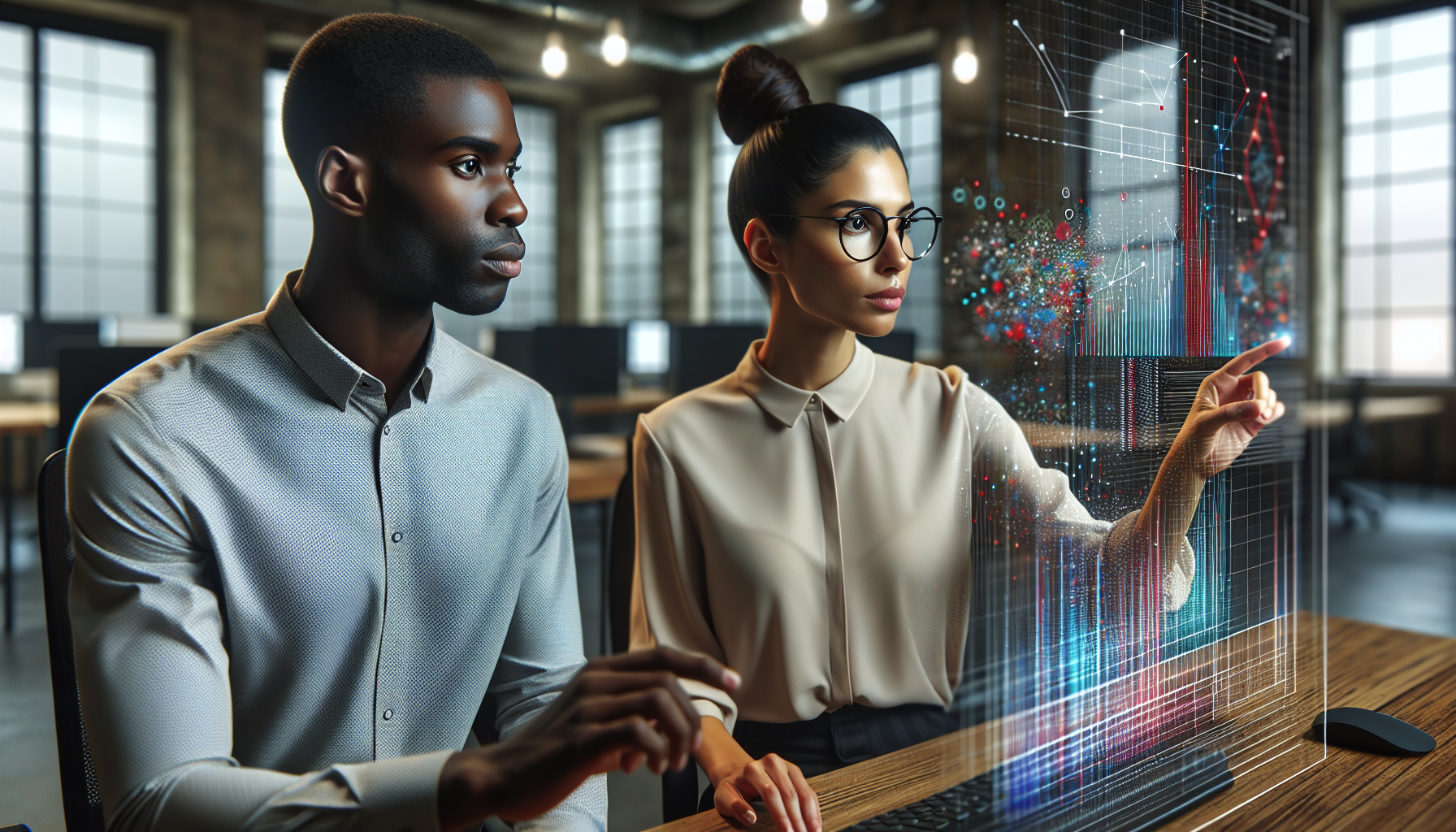
What is predictive analytics?
Predictive analytics is the use of data, statistical algorithms, and machine learning techniques to identify the likelihood of future outcomes based on historical data.
How does predictive analytics improve marketing?
It allows marketers to anticipate customer needs, personalize marketing efforts, and optimize resource allocation for better ROI.
What are the challenges of using predictive analytics in marketing?
Challenges include data integration, privacy concerns, and the need for continuous adaptation to new technologies.
Can small businesses benefit from predictive analytics?
Yes, small businesses can leverage predictive analytics to compete more effectively by targeting their marketing efforts more precisely and efficiently.
What tools are used in predictive analytics?
Common tools include R, Python, SAS, and IBM SPSS Modeler, which help in building and refining predictive models.
Future Predictions
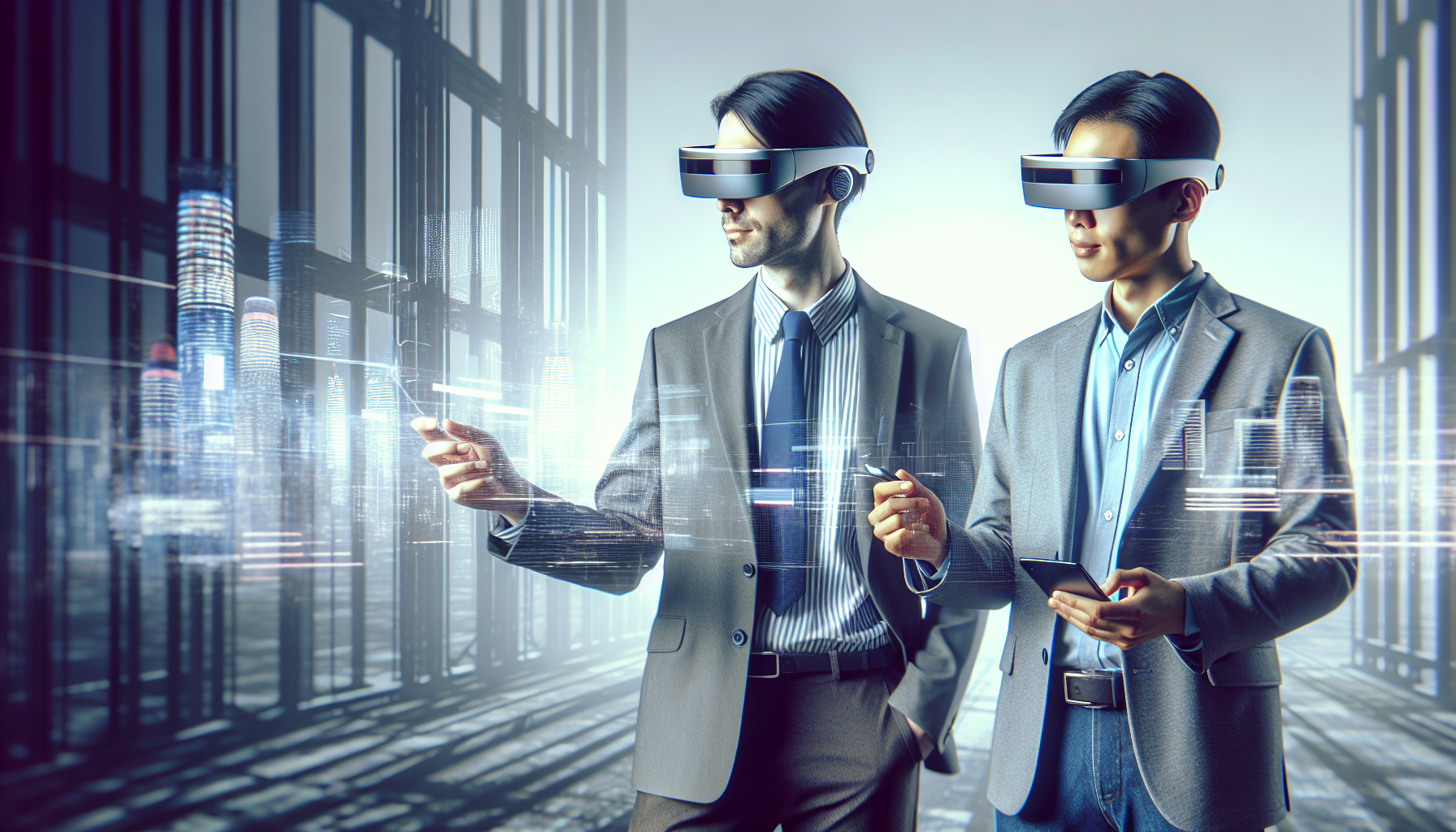
As we look to the future, predictive analytics is set to become even more integral to marketing strategies. Here are five predictions:
- Increased Personalization: Marketing strategies will become more personalized, using predictive analytics to tailor content to individual preferences and behaviors.
- Greater Integration with AI: Predictive analytics will increasingly integrate with AI technologies, enhancing the automation and accuracy of marketing campaigns.
- Expansion in Small Business Use: As tools become more accessible and affordable, more small businesses will adopt predictive analytics.
- Enhanced Privacy Measures: As privacy concerns grow, predictive analytics tools will evolve to provide more secure and privacy-compliant solutions.
- Advancements in Real-Time Analytics: Real-time data processing will become more prevalent, allowing marketers to adjust campaigns instantly based on user interactions.
References
- Predictive Analytics in Marketing: 7 Use Cases – A comprehensive guide on how predictive analytics is applied in marketing.
- Predictive analytics in marketing: everything you need to know – An article that covers the basics and importance of predictive analytics in marketing.
- How to Use Predictive Analytics in Data-Driven Marketing – A detailed exploration of the role of predictive analytics in modern marketing strategies.
- What Is Predictive Advertising & Why Do You Need It? – An article explaining the concept and benefits of predictive advertising.
Disclaimer
This article is AI-generated for educational purposes and does not intend to give advice or recommend its implementation. It aims to inspire readers to research and delve deeper into the topics covered.